Career in Data Science: In today’s data-driven world, data science stands as a mighty force, poised to revolutionise industries and reshape our lives. If you’re starting your career or exploring a future-proof profession, a career in data science offers exciting options. It decodes fraud mysteries, transforms healthcare, and customises internet searches, ads, and gaming. If you want to pursue a profitable career in data science, a Full-Stack Data Science course can greatly help you. In this blog, we’ll tell you about 10 domains that would be best for people starting a career in data science.
Top 10 Domains for Starting a Career in Data Science
Here are the 10 best domains for starting a career in data science in:
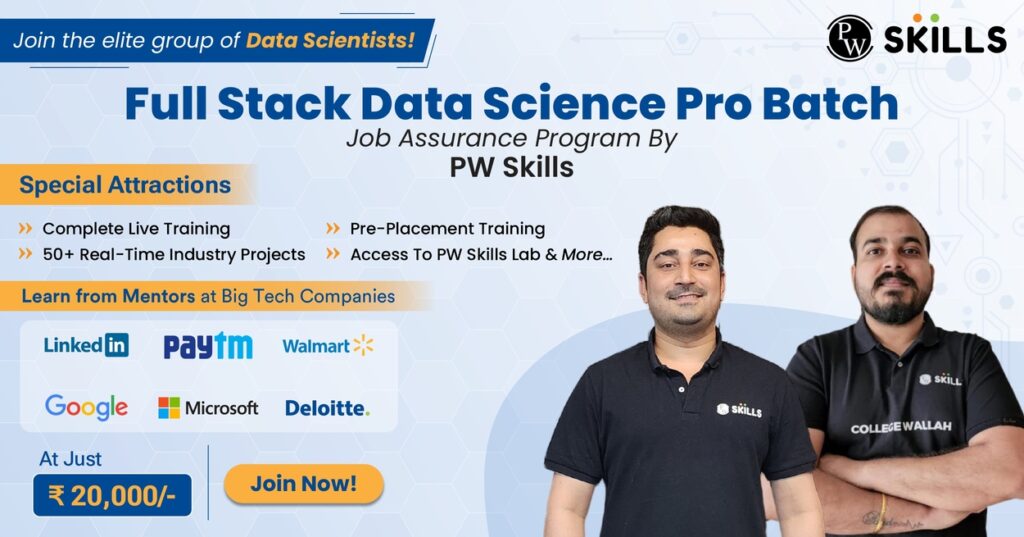
1. Fraud and Risk Detection
Fraud detection is a vital use of data science in many sectors, as it thwarts financial losses and safeguards businesses and customers. Let’s delve into data science’s significance in fraud detection in various domains:
- Financial Sector: In finance, data science is pivotal for spotting credit card fraud, detecting unusual transactions, and reducing unauthorised access risk. Advanced machine learning algorithms can identify suspicious activities in real-time by analysing transaction history and customer behaviour.
- E-commerce: Online businesses depend on data science to identify fraudulent orders. They analyse customer behaviour and transaction history to differentiate between legitimate and fraudulent purchases, protecting against credit card fraud and payment scams.
- Insurance: Data science is essential in the insurance sector for identifying fraudulent claims and assessing risk. Data analysis and machine learning detect inconsistencies in claims, such as frequent claims for the same medical procedure or suspicious provider behaviour, saving costs and upholding service integrity.
Data science also plays a critical role in risk management, specifically in:
- Credit Scoring: Lenders employ data science to assess creditworthiness by analysing financial data and transaction patterns, ensuring informed loan decisions.
- Insurance Underwriting: Insurers assess risk profiles by analysing individual or business data, setting appropriate premiums and coverage levels for fair pricing.
- Supply Chain Management: Data science aids organisations in monitoring and mitigating risks by analysing supply chain data and using predictive analytics to ensure a smooth flow of goods and services.
2. Healthcare
The healthcare field has undergone a significant transformation in the era of data science. Electronic health records (EHRs), big data analytics, and advanced machine learning have made data science invaluable in healthcare.
- Data Science Applications in Healthcare
Electronic Health Records (EHRs)
EHRs have revolutionised patient data management. Data scientists use techniques like data mining and natural language processing to extract insights from patient histories, diagnoses, and treatment plans. This enhances healthcare coordination, reduces errors, and optimises treatment.
Predictive Analytics
Data scientists use historical data to predict health scenarios, such as readmissions and disease outbreaks. Machine learning identifies high-risk patients, enabling proactive care.
Drug Discovery
Pharmaceutical companies employ data science to expedite drug discovery. This reduces costs and identifies candidates for clinical trials, bringing drugs to patients faster.
- Predictive Analytics for Disease Management
Early Diagnosis
Data science, including deep learning and computer vision, aids in early detection of conditions like cancer, cardiovascular diseases, and neurological disorders through medical imaging analysis.
Personalized Treatment Plans
Data-driven algorithms tailor treatment plans based on individual medical history and genetic information, improving effectiveness and reducing side effects.
Resource Allocation
Predictive analytics ensures healthcare facilities are adequately staffed and equipped by analysing historical data and patient trends.
- Improving Patient Care with Data-Driven Insights
Remote Monitoring
Data science and IoT analyse patient data from wearable devices, triggering alerts to healthcare providers for timely interventions in managing chronic conditions.
Preventive Medicine
Population-level health data analysis identifies at-risk communities and enables the development of preventive healthcare programs, reducing disease incidence.
Patient Engagement
Data science tools like patient portals and mobile apps enhance communication between healthcare providers and patients, promoting informed decision-making and better health outcomes.
Also check: Data Science for Beginners
3. Internet Search
In the age of the internet’s central role in our daily lives, search engines are crucial tools for accessing information. Complex algorithms and data science techniques underlie these search engines, making the internet more accessible and useful. We will explore how data science plays a vital role in internet search and impacts the content we find online.
- Search Engines and Data Science
Backbone of Search: Google, Bing, and Yahoo are the initial stops for people seeking information online. Data science is the foundation of these search engines, efficiently managing vast web data.
- Data Processing: Data scientists create systems to process and index web pages. They design algorithms to gather and assess web content for search engines to provide high-quality results.
- Scalability: Data science ensures search engines can handle billions of web pages. Techniques are developed to swiftly and accurately crawl and index immense amounts of data.
- Web Crawling and Indexing: Fundamental processes in search engine operation.
- Web Crawling: Systematic exploration of the web, guided by efficient crawling algorithms to prioritise and frequent crawling of pages.
- Indexing: Data scientists develop algorithms to create a database of web content, including keywords, metadata, and links, to respond to user queries.
- Ranking Algorithms: The heart of search engines, determining the order of search results, developed with data science.
- Keyword Relevance: Mathematical models analyse keyword relevance to user queries, factoring in frequency and position.
- User Behaviour: Data scientists use user behaviour data like click-through rates to determine search result satisfaction.
- Quality Assessment: Data science evaluates web page quality, considering factors such as load speed, security, and trustworthiness.
- Personalized Search
Understanding User Behaviour: Data science is instrumental in understanding user behaviour, enhancing search results and user satisfaction.
- Search Queries: Data science analyses query patterns, detecting variations, misspellings, and intent.
- Click-Through Rates (CTR): Monitoring user click choices and time spent on pages informs user satisfaction.
- Dwell Times: Data scientists assess how long users stay on pages, indicating relevance and engagement.
- Personalised Recommendations: Data-driven algorithms provide user-specific suggestions, enriching the user experience.
- User Profiles: Data science creates user profiles using search history and contextual data for tailored results.
- Behavioural Analysis: Patterns, interests, and trends identified in behavioural analysis guide personalised content recommendations.
- Content Recommendations: Search engines offer content recommendations based on user preferences and history.
- The Challenge of Privacy: Personalised search raises privacy concerns.
- Data Protection: Data scientists adhere to strict privacy regulations, employing anonymization techniques and secure storage.
- Opt-In Features: Users can choose to enable personalised search features, maintaining greater control over their privacy.
- The Impact of Data Science on Online Information Retrieval
- Evolving Search Technology: Data science drives the ongoing evolution of search engines.
- Voice Search: Data science enables voice search, allowing users to speak their queries.
- Image Search: Advanced image recognition and data science facilitate image-based search.
- Semantic Search: Data science enhances search engines’ understanding of context and semantics, improving the interpretation of complex queries.
- Natural Language Processing: Data science uses NLP techniques to better understand words and phrases in queries.
- Sentiment Analysis: Data science determines the sentiment behind search queries, resulting in more accurate results.
- Future Developments: Expect exciting advancements in internet search.
- Machine Learning: Incorporating machine learning and deep learning enhances result accuracy.
- User Intent Prediction: Data science will improve user intent prediction for more relevant results.
- Real-Time Search: Advancements allow real-time data retrieval from social media and news sources.
4. Targeted Advertising
In the realm of modern advertising, precise audience targeting is crucial for successful marketing. Data science plays a pivotal role in this. Let’s explore how it’s changing the way brands connect with their customers.
- Data Science’s Impact on Advertising
- Customer Segmentation: Data science dissects audiences into detailed segments. Marketers analyse vast datasets to identify customer groups by demographics, interests, and behaviour. This helps tailor ads for specific customer personas, making them more relevant.
- Predictive Analytics: Predictive analytics forecasts customer behaviour and preferences. Machine learning predicts what products or services might interest a customer, allowing precise targeting.
- Dynamic Content Optimization: Data-driven platforms customise ad content in real time based on user profiles. Ad creative, copy, and formats adapt to maximise relevance and click-through rates.
- Personalized Ad Targeting
- Retargeting: Data science enables retargeting ads to users who have previously engaged with a brand. These serve as reminders and effectively convert potential customers into buyers.
- Lookalike Audiences: Advertisers use data science to create “lookalike audiences” with characteristics similar to existing customers, expanding their reach.
- Behavioural Targeting: Tracking user online activities and tailoring ads based on behaviour is called behavioural targeting. Data science assesses clicks, searches, and browsing history for relevant ad placements.
- Measuring Ad Campaign Effectiveness
- A/B Testing: Data science conducts A/B tests to compare different ad variations and optimise campaign performance. Testing elements like headlines, images, and calls-to-action helps identify what resonates with the audience.
- Conversion Rate Optimization: Data science tracks conversion rates and analyses factors influencing them, aiding informed campaign adjustments.
- ROI Analysis: Data science calculates the return on investment (ROI) for advertising campaigns. This tracks revenue generated in comparison to ad spend, aiding effective budget allocation.
5. Road Travel
- Data Science in the Automotive Industry
The auto industry has undergone a major transformation with data science integrated into vehicle technology. Data science enhances road travel safety, efficiency, and overall experience. Here’s how:
- Autonomous Vehicles: Self-driving cars, known as autonomous vehicles, rely on data science for navigation. They process real-time data from sensors, cameras, lidar, and radar to understand their surroundings and make driving decisions.
- Telematics: Telematics systems use data science to monitor driving behaviour, vehicle performance, and fuel efficiency. Insurers use this data for personalised insurance rates based on individual driving habits.
- Autonomous Vehicles and Data-Driven Decisions
- Data Collection: Autonomous vehicles gather data through sensors and cameras, monitoring road conditions, other vehicles, pedestrians, and obstacles, as well as traffic signals and signs.
- Data Processing: Advanced data science algorithms and machine learning models process collected data in real-time, enabling decisions like changing lanes, slowing down, accelerating, or stopping in potential danger situations.
- Safety: Data science predicts and prevents accidents in autonomous vehicles. Data-driven collision avoidance systems react faster than human drivers, enhancing safety.
- Efficiency: Data-driven decisions optimise routes, reduce fuel consumption, and minimise emissions in autonomous vehicles, promoting eco-friendly travel.
- Traffic Prediction and Optimization
Data science benefits traffic flow and congestion management in urban areas.
- Traffic Prediction: Data science algorithms analyse historical traffic data, weather conditions, and events to predict congestion. Mobile apps and navigation systems use these predictions to offer real-time traffic updates and alternative routes to minimise travel time.
- Traffic Signal Optimization: Smart traffic signal systems employ data science to adjust signal timing based on traffic patterns, reducing jams, travel times, and fuel consumption.
- Reducing Emissions: Data-driven traffic management and congestion reduction lower emissions and support environmental sustainability by reducing idling time and unnecessary fuel use.
6. Government
Data science’s role in government has grown, aiding agencies in data-driven advancements. They utilise data to improve services, enhance decision-making, and tackle societal issues. Let’s delve into data science’s applications in government and its importance.
- Government’s Data Science Applications
- Predictive Policing: Law enforcement uses data science for crime prediction. They analyse historical crime data, geographic information, and other factors to foresee high-risk areas and times for criminal activities, thus enhancing resource allocation and reducing crime.
- Healthcare Management: Agencies like the CDC and WHO use data science to monitor public health trends, track disease outbreaks, and allocate resources during health crises. Data analytics play a pivotal role in pandemic response and vaccination distribution.
- Education: Data science assesses student performance, improving educational outcomes and resource allocation. Analysis identifies struggling students for targeted interventions and better educational experiences.
- Data-Driven Public Service Enhancement
- Smart Cities: Governments transition to “smart cities” using data science to optimise urban infrastructure, from traffic management to energy efficiency. Sensors in streetlights monitor traffic flow, reducing congestion.
- Social Services: Social agencies use data science to identify those in need. Data analysis enhances resource allocation for welfare, housing assistance, and food support, ensuring the most vulnerable receive aid.
- Economic Development: Data-driven strategies foster economic growth. Identifying key industries and analysing market trends guides investments to stimulate local economies.
- Data-Driven Policy Making
- Evidence-Based Decision Making: Data science guides evidence-based policymaking. Policymakers rely on data-driven insights for effective policies that meet public needs.
- Transparency and Accountability: Government data availability promotes transparency and accountability. Citizens access and analyse data, holding officials accountable and ensuring efficient resource use.
- Disaster Response and Mitigation: During disasters, data science aids in response and mitigation. Real-time data and satellite imagery predict and respond to events like hurricanes, floods, and wildfires effectively.
7. Website Recommendations
- The Significance of Data Science in Recommendation Systems:
In the digital era, online platforms heavily rely on recommendation systems. When you shop on Amazon, stream content on Netflix, or browse social media, these platforms consistently offer suggestions to keep you engaged. Data science is pivotal in crafting these recommendation systems.
Data scientists employ diverse methods like collaborative filtering, content-based filtering, and deep learning to analyse user preferences and behaviour. By comprehending user likes and dislikes, these systems provide tailored product, movie, music, or content recommendations. The algorithms they use not only enhance user satisfaction but also drive business revenue.
- Netflix and Amazon’s Data-Driven Content Suggestions:
Let’s explore Netflix and Amazon as examples of how data science powers recommendation systems:
- Netflix: This platform utilises a complex recommendation algorithm that hinges on user interaction data, including viewing history, viewing times, and genre, actor, and director preferences. They continuously update this data to refine and enhance recommendations, ensuring viewer satisfaction.
- Amazon: Amazon’s recommendation engine is renowned for suggesting products aligned with your interests. It analyses your past purchases, browsing history, and the choices of customers with similar preferences. This data-driven approach enhances the customer experience and boosts sales.
- Crafting Personalised Recommendation Engines:
Data scientists specialising in recommendation systems need a solid grasp of machine learning, data mining, and natural language processing. They work with vast datasets, fine-tune algorithms, and use real-time user feedback to enhance accuracy. For those entering the data science field, mastering recommendation systems holds great promise, as they are prevalent across various industries, from e-commerce to content streaming.
8. Advanced Image Recognition
- The Fascination of Image Recognition in Data Science
Image recognition, a subset of computer vision, stands out as an enthralling area within data science. It involves teaching machines to understand and interpret images, enabling them to identify objects, individuals, and even emotions. This technology has diverse applications, from healthcare to security.
Data scientists employ convolutional neural networks (CNNs) and deep learning techniques to train models for image recognition. These models learn patterns and features within images, enabling them to perform tasks like facial recognition, object detection, and image classification.
- Applications in Healthcare, Security, and Beyond
- Healthcare: Image recognition transforms medical diagnostics by identifying diseases, anomalies in medical images (like X-rays and MRIs), and tracking patient vital signs.
- Security: Surveillance systems use image recognition to detect and alert unusual activities, and facial recognition technology has applications in security and access control.
- Retail: Retailers utilise image recognition for inventory monitoring, streamlined checkout processes, and personalised shopping experiences.
- Challenges and Prospects in Image Recognition
Image recognition, while promising, faces challenges such as the need for extensive and diverse training datasets and addressing algorithmic bias. Nevertheless, it continues to advance, with exciting prospects including improved accuracy, real-time processing, and new applications.
9. Speech Recognition
- The Wonders of Speech Recognition Technology
Speech recognition, also known as automatic speech recognition (ASR), is a captivating subfield of data science. It involves converting spoken language into text, enabling voice-controlled applications and enhancing customer service interactions.
ASR technology employs machine learning models, including deep neural networks, to recognize and transcribe spoken language. It finds use in various applications, from voice assistants like Siri and Alexa to transcription services and call centre analytics.
- Voice Assistants and Customer Service
Voice assistants have become ubiquitous in modern life, used for tasks, questions, and controlling smart devices. Behind these applications are sophisticated ASR models that understand and respond to spoken commands.
In customer service, speech recognition automated call routing, generates insights from customer interactions, and ensures consistent, accurate responses.
- Enhancing Speech Recognition Accuracy
Data scientists in speech recognition work to enhance model accuracy, reduce error rates, and improve recognition of different accents and languages. They also focus on real-time processing and creating more natural-sounding synthesised speech.
10. Gaming
- Data Science Revolutionising the Gaming Industry
Data science has transformed the gaming industry. Data scientists utilise player data to enhance the gaming experience, create personalised content, and improve game design.
They collect and analyse data on player behaviour, preferences, and interactions within the game. This data informs decisions on game mechanics, level design, in-game purchases, and more.
- Player Behavior Analysis and Game Development
Data scientists use player behaviour data to understand how gamers engage with a game, tracking challenging levels, popular items, and player progression. This information guides developers in enhancing gameplay and user engagement.
Recommended Course
- Decode DSA with C++
- Full Stack Data Science Pro Course
- Java For Cloud Course
- Full Stack Web Development Course
- Data Analytics Course
Conclusion
Data science offers limitless opportunities in our data-driven world. It safeguards against fraud and transforms healthcare. It tailors recommendations and enhances gaming. It impacts every aspect of our lives. Embrace this dynamic field to shape your future.
Enrol in the PW Skills Full Stack Data Science Pro course today and invest in your future. With our job assurance program, you can be confident that you’ll be ready for a successful career in data science.