Data science vs. cyber security is a crucial comparison in the tech world. While data science focuses on extracting insights from data, cyber security is about protecting systems. Both fields play pivotal roles in today’s digital landscape, with data scientists driving innovation and cyber security professionals safeguarding against cyber threats.
It is within this dynamic interplay of innovation and safeguarding that we explore the realms of data science and cyber security. Hence, in this blog, we’ll compare data science vs cyber security and tell you everything there is to know about these 2 fields!
If you want to secure a high-paying job as a data scientist, a Full-Stack Data Science course is highly recommended!
What Is Cyber Security?
Cyber Security is the practice of safeguarding computer systems, networks, and data from unauthorised access, attacks, and damage. It employs technologies, processes, and practices to ensure the confidentiality, integrity, and availability of digital information in today’s interconnected landscape.
Fundamental Concepts
At its heart, cyber security relies on core ideas ensuring digital info’s confidentiality, integrity, and availability. Encryption, access controls, and authentication bolster the digital infrastructure against malicious actors exploiting weaknesses.
Evolution of Cyber Threats
The landscape of cyber threats is dynamic and continually morphing. Cyber threats have evolved from early viruses to advanced attacks like ransomware and DDoS assaults. Cyber security experts must grasp this evolution to effectively combat emerging threats.
Necessity for Robust Security Measures
With our growing dependence on digital platforms, the demand for strong security measures rises. This section highlights the rising frequency and complexity of cyber-attacks, stressing the need for organisations and individuals to adopt thorough cyber security strategies. Security breaches, affecting entities from financial institutions to healthcare providers, can lead to severe consequences, including financial losses and the compromise of sensitive personal information.
Protecting Critical Infrastructure
Essential systems like power grids, transportation, and communication networks face serious cyber threats. Safeguarding them relies heavily on cybersecurity, crucial for averting catastrophic consequences that can impact entire societies.
Also Check: Top 15+ Cyber Security Books to Read in 2023
What Is Data Science?
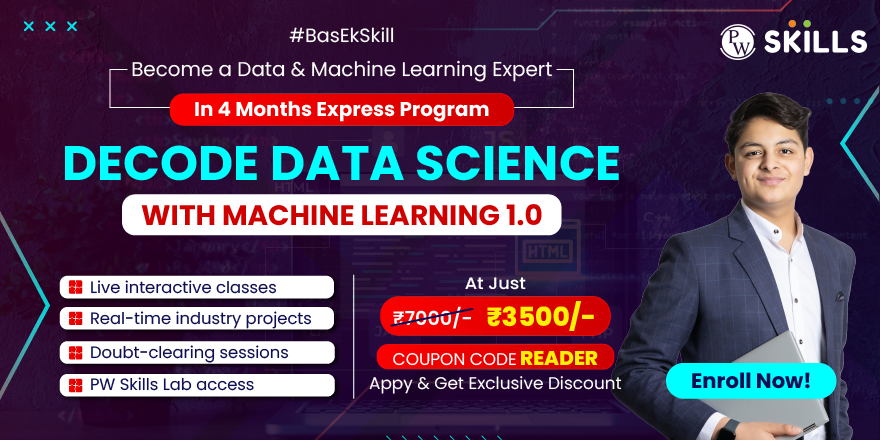
Data Science is a multidisciplinary field that employs scientific methods, processes, algorithms, and systems to extract insights and knowledge from structured and unstructured data. It combines expertise in statistics, mathematics, and programming to interpret complex information for informed decision-making.
Foundations of Data Science
- Statistics and Mathematics: Statistical principles form the bedrock of data science, enabling professionals to identify patterns, make predictions, and derive meaningful conclusions from data. Mathematical concepts, such as linear algebra and calculus, are pivotal in developing algorithms and models.
- Computer Science: Data science heavily relies on programming languages like Python, R, and Julia. Proficiency in coding is crucial for tasks ranging from data cleaning and preprocessing to the development of machine learning models.
Data Science Lifecycle
- Data Collection: The process begins with the collection of vast amounts of raw data from diverse sources, including sensors, social media, and enterprise databases.
- Data Cleaning and Preprocessing: Raw data is often messy and incomplete. Data scientists engage in cleaning and preprocessing to ensure that the data is accurate, consistent, and ready for analysis.
- Exploratory Data Analysis (EDA): EDA involves visualising and understanding the patterns and relationships within the data. This step is crucial for formulating hypotheses and refining the focus of subsequent analyses.
- Model Development and Training: Machine learning models are crafted and trained using algorithms to make predictions or uncover patterns within the data.
- Evaluation and Validation: The developed models are rigorously evaluated to ensure accuracy and effectiveness. Validation ensures that the model generalises well to new, unseen data.
- Deployment and Maintenance: Successful models are deployed into real-world applications, and ongoing maintenance is essential to adapt to evolving data patterns.
Applications of Data Science
- Business Intelligence: Data science empowers organisations to make informed decisions by providing insights into market trends, customer behaviour, and operational efficiency.
- Healthcare: From predicting disease outbreaks to personalised medicine, data science is revolutionising healthcare by leveraging patient data for more accurate diagnoses and treatment plans.
- Finance: Financial institutions utilise data science for risk management, fraud detection, and algorithmic trading to gain a competitive edge in the market.
- E-commerce and Marketing: Recommender systems, targeted advertising, and customer segmentation are just a few ways data science enhances the efficacy of e-commerce and marketing strategies.
- Technology and Innovation: Data science fuels innovation by uncovering patterns that drive advancements in artificial intelligence, robotics, and other cutting-edge technologies.
Read more: Data Science Applications Used in Daily Life in 2023
How Are Cyber Security and Data Science Interrelated?
The interrelation between cyber security and data science marks a pivotal synergy in the contemporary digital landscape. As technology evolves, so do the methods employed by malicious actors, necessitating advanced strategies for threat detection, prevention, and mitigation.Â
Data-Driven Threat Intelligence
In the realm of cyber security, threat intelligence plays a central role in identifying and understanding potential risks. Data science, with its powerful analytical tools and algorithms, aids in the systematic analysis of vast datasets to discern patterns indicative of cyber threats. This proactive approach enhances the ability to predict and counteract potential security breaches.
Behavioural Analytics for Anomaly Detection
One of the key challenges in cyber security is identifying anomalous activities that may signify a security breach. Data science, particularly through the application of behavioural analytics, allows for the creation of models that establish a baseline of normal behaviour. Deviations from this baseline can then be flagged as potential security incidents, enabling rapid response and mitigation.
Machine Learning in Intrusion Detection Systems (IDS)
Intrusion Detection Systems are fundamental to cyber security, and machine learning algorithms contribute significantly to their efficacy. By leveraging historical data and continuously learning from new patterns, machine learning algorithms within IDS become adept at recognizing and thwarting novel cyber threats in real-time.
Predictive Analysis for Vulnerability Management
Predictive analysis, a core component of data science, is employed to forecast potential vulnerabilities in a system. By analysing historical data on security incidents, combined with ongoing monitoring, predictive analytics helps organisations pre-emptively address weaknesses, fortifying their cyber defences.
Cybersecurity Data Science in Incident Response
In the aftermath of a cyber-attack, swift and accurate incident response is critical. Cybersecurity data science aids in post-incident analysis by examining log data, network traffic, and other relevant information. This retrospective analysis not only helps understand the nature of the attack but also strengthens defences against similar future threats.
Adaptive Authentication and Fraud Detection
With the rise of sophisticated cyber threats, traditional authentication methods often fall short. Data science introduces adaptive authentication, which dynamically assesses user behaviour and context to determine the authenticity of access attempts. This approach enhances security by adapting to evolving patterns and recognizing potential fraud in real-time.
Continuous Monitoring and Proactive Defense
Cyber threats are dynamic and ever-evolving. Data science facilitates continuous monitoring of networks and systems, allowing for real-time threat detection and response. This proactive defence strategy, informed by data-driven insights, is crucial in staying ahead of the constantly changing threat landscape.
A Word About Cybersecurity Data Science
In the rapidly evolving landscape of cybersecurity, the fusion of data science techniques has given rise to a specialised field known as cybersecurity data science.Â
What Is Cybersecurity Data Science?
In the realm of cybersecurity, data science plays a key role. This interdisciplinary field combines data science principles with the unique challenges presented by cybersecurity threats. Essentially, it uses advanced analytics, machine learning, and statistical models to identify patterns, anomalies, and potential risks within extensive and intricate datasets.
The Role of Data Analytics in Identifying Threats
One of the primary applications of cybersecurity data science is in the realm of threat detection and analysis. By applying sophisticated data analytics, cybersecurity professionals can sift through massive datasets generated by network logs, user activities, and system behaviours. Through this process, they can identify unusual patterns that may indicate potential security breaches, intrusions, or malicious activities.
Behavioural Analysis and Anomaly Detection
Cybersecurity data science places a strong emphasis on behavioural analysis and anomaly detection. By establishing baselines for normal system behaviour, any deviation from these patterns can be flagged as potential security threats. Machine learning models play a crucial role in learning and adapting to evolving cyber threats, enabling systems to recognize novel attack vectors and tactics.
Proactive Threat Mitigation
One of the key advantages of cybersecurity data science is its ability to enable proactive threat mitigation. Traditional cybersecurity approaches often rely on reactive measures, responding to incidents after they occur. In contrast, cybersecurity data science empowers organisations to anticipate and prevent potential threats by identifying vulnerabilities, predicting attack vectors, and implementing pre-emptive measures.
Challenges and Opportunities
While cybersecurity data science offers tremendous potential, it also presents unique challenges. The field requires a deep understanding of both cybersecurity principles and advanced data science techniques. Moreover, the ever-changing nature of cyber threats necessitates continuous adaptation and innovation.
Integration with Cybersecurity Operations
In practice, cybersecurity data science is seamlessly integrated into cybersecurity operations. It collaborates with firewalls, antivirus programs, and intrusion detection systems. Data science insights aid better decision-making for staying ahead of cyber adversaries.
The Future of Cybersecurity Data Science
As cyber threats become more sophisticated and pervasive, the role of cybersecurity data science is poised to expand. The field will likely continue to evolve with advancements in artificial intelligence, automation, and predictive analytics, providing organisations with robust tools to defend against an ever-changing threat landscape.
 The Significance and Uses of Cyber Security
As cyber threats grow in complexity and reach, cybersecurity data science’s role is set to grow. The field will probably advance with AI, automation, and predictive analytics, offering strong tools against a changing threat landscape. This section delves into the multifaceted importance and diverse applications of cyber security.
Protecting Sensitive Information
Cybersecurity plays a pivotal role in securing sensitive data. From personal information stored in databases to financial transactions conducted online, the confidentiality of such data is paramount. Robust cybersecurity measures, including encryption and access controls, are essential safeguards against unauthorised access and data breaches.
Safeguarding National Security
Cyber-attacks pose a threat to national security, targeting government agencies, military institutions, and critical infrastructure like power grids. Effective cybersecurity is vital to prevent widespread disruptions.
Preventing Financial Losses
Financial repercussions hit businesses and individuals hard through activities like ransomware and fraud, causing significant losses. Cybersecurity serves as a financial defence, blocking unauthorised access, securing financial transactions, and thwarting fraudulent actions.
Ensuring Business Continuity
Maintaining business continuity is paramount for organisations facing cyber threats. Cybersecurity measures, including backup systems and disaster recovery plans, are crucial to minimise the effects of attacks and ensure smooth operations, even after a cyber incident.
Preserving Trust and Reputation
The trust of customers, clients, and stakeholders is vital for any organisation. A breach of security not only jeopardises sensitive information but also erodes trust. Cybersecurity, by preventing and mitigating security incidents, plays a crucial role in preserving the reputation and trustworthiness of businesses and institutions.
Adapting to Evolving Threats
The digital landscape is dynamic, with cyber threats constantly evolving. Cybersecurity professionals are at the forefront of adapting to new threats, developing proactive measures to anticipate and counteract emerging risks. This adaptability is crucial in maintaining effective defence against the ever-changing tactics of cyber adversaries.
International Collaboration in Cybersecurity
In an interconnected world, cyber threats transcend national borders. International collaboration in cybersecurity is essential for sharing threat intelligence, coordinating responses to cyber incidents, and establishing global standards for cyber hygiene. Cybersecurity efforts are integral to fostering a secure and collaborative digital ecosystem on a global scale.
The Significance and Uses of Data Science
In the ever-expanding realm of digital information, data science emerges as a transformative force, wielding the power to extract valuable insights from vast and complex datasets. This section delves into the multifaceted significance and diverse applications of data science across industries.
Understanding Data Science’s Significance
Data science serves as the linchpin in the era of information overload. By employing advanced statistical and mathematical techniques, data scientists sift through large datasets to discern patterns, trends, and actionable insights. Businesses, government agencies, and research institutions leverage these insights to make informed decisions, optimise processes, and gain a competitive edge.
Applications in Business Intelligence
One of the primary applications of data science lies in business intelligence. Through the analysis of customer behaviour, market trends, and operational metrics, organisations can tailor their strategies, refine marketing efforts, and enhance customer experiences. Data-driven decision-making becomes a strategic advantage, enabling businesses to pivot swiftly in response to dynamic market conditions.
Driving Innovation across Industries
Beyond business applications, data science fuels innovation across various sectors. In healthcare, predictive analytics based on patient data can improve diagnosis and treatment plans. In finance, data-driven algorithms enhance risk management and investment strategies. The intersection of data science with fields like agriculture, energy, and transportation opens new frontiers for efficiency and sustainability.
Optimising Operational Processes
Data science is instrumental in streamlining operational processes. Through predictive maintenance models, industries can pre-emptively address equipment failures, minimising downtime and maintenance costs. Supply chain optimization, fraud detection, and inventory management are additional areas where data science interventions yield tangible benefits.
Enabling Personalised Experiences
The significance of data science is perhaps most palpable in the realm of personalised experiences. From recommendation engines on streaming platforms to personalised product recommendations in e-commerce, data science algorithms analyse user behaviour to deliver tailored content and services. This not only enhances user satisfaction but also contributes to increased engagement and brand loyalty.
Challenges and Ethical Considerations
Despite its transformative potential, data science is not without challenges. Issues related to data privacy, bias in algorithms, and ethical considerations surrounding the use of personal information require careful navigation. This section delves into the responsibility of data scientists to address these challenges, emphasising the importance of ethical practices in the field.
Cyber Security vs. Data Science Career Paths and Growth
- mitigating security incidents, minimising potential damage.
- Security Consultants: Providing expert advice, consultants assess security postures, recommend improvements, and aid in the implementation of robust security measures.
- Security Architects: These professionals design comprehensive security systems, considering the organisation’s specific needs and potential threats.
Unravelling Data Science Career Paths
- Data Analysts: Entry-level positions involve collecting, processing, and analysing data to extract meaningful insights that inform decision-making.
- Machine Learning Engineers: These professionals work on developing and implementing machine learning algorithms to improve systems and processes.
- Data Scientists: Central to the field, data scientists leverage statistical analysis and machine learning to derive actionable insights from complex datasets.
- Data Architects: Responsible for designing and managing an organisation’s data infrastructure, architects ensure the efficient flow and storage of data.
Data Science vs. Cyber Security: Which Pays More?
When contemplating a career path, one often considers financial rewards. In the realm of data science and cyber security, compensation is influenced by various factors. Data scientists, equipped with skills in machine learning, statistical analysis, and programming, often command competitive salaries. The demand for these professionals continues to rise as organisations recognize the value of data-driven decision-making.
On the other side of the spectrum, cyber security professionals are equally well-compensated. With the persistent threat of cyber-attacks, businesses are investing heavily in securing their digital assets. Cybersecurity experts, specialising in areas such as ethical hacking, incident response, and risk management, find themselves in high demand, translating into attractive remuneration packages.
Also Read: Data Security in 2023
Data Science vs. Cyber Security: Which Is Easier?
The question of ease in choosing between data science and cyber security is subjective and contingent on individual strengths, interests, and learning preferences. Data science, with its focus on mathematics, statistics, and programming, can be challenging for those without a strong quantitative background. However, the creative aspect of deriving insights from data appeals to those with a passion for problem-solving and curiosity.
Conversely, cyber security demands a keen understanding of networks, system vulnerabilities, and the ability to stay ahead of constantly evolving threats. For individuals with a penchant for detective work, ethical hacking, and an analytical mindset, cyber security may feel more intuitive. The dynamic nature of both fields requires continuous learning, making the journey challenging yet rewarding.
Ultimately, the perceived difficulty lies in alignment with personal strengths and interests. Whether one finds joy in unravelling data patterns or thrives on securing digital fortresses, the “ease” of the chosen path is a highly individualised experience. In the dynamic landscape of technology, individuals are often faced with the pivotal choice between pursuing a career in cyber security or data science. Understanding the unique trajectories and growth opportunities in each field is crucial for those contemplating their professional journey.
Exploring Cyber Security Career Paths
- Security Analysts: Entry-level roles often involve monitoring an organisation’s networks for security breaches and implementing preventive measures.
- Incident Responders: Professionals in this role focus on swiftly addressing andÂ
Conclusion
In the digital convergence of data science and cyber security, a symbiotic dance unfolds. Cybersecurity safeguards, while data science empowers. It’s not a choice but an invitation to explore their intertwined realms, offering opportunities for growth and innovation. Embrace the complexities and step into a resilient, data-driven future.
Are you passionate about solving real-world problems with data? If so, then a career in data science is the perfect fit for you. With the PW Skills Full Stack Data Science Pro course, you’ll learn how to use data to make informed decisions and solve real-world problems in a variety of industries. Make a difference in the world and enroll in our course today!
FAQs
What role do ethical considerations play in cybersecurity decision-making?
Ethical considerations are paramount in cybersecurity decision-making, guiding the responsible use of technology, transparent communication, and the protection of user privacy.
How do cybersecurity professionals stay ahead of emerging cyber threats?
Cybersecurity professionals stay ahead by continuous learning, attending industry conferences, participating in threat-sharing communities, and engaging in simulations to anticipate and respond to evolving threats.
Can data science be used for proactive cybersecurity risk management?
Yes, data science enables proactive cybersecurity risk management by identifying potential vulnerabilities, predicting attack vectors, and guiding the allocation of resources for optimal defence.
Do advancements in quantum computing pose new challenges to cybersecurity?
Yes, the advent of quantum computing presents new challenges, but it also prompts cybersecurity professionals to develop quantum-resistant encryption and security measures to adapt to this evolving landscape.
How does the human element factor into cybersecurity strategies?
Recognizing the human element is crucial in cybersecurity, as education, awareness training, and user behaviour analysis play pivotal roles in fortifying defences against social engineering and insider threats.