Data Science in Finance is an essential part of the banking industry nowadays. The increasing adoption of data science in organizational and decision-making processes has led to a high demand for data scientists across various sectors.Â
Data Science in Finance: As technology continues to drive economic modernization, data science in finance is playing an increasingly critical role in transforming the world of banking and investment. Enabling faster, more accurate decisions with customer experience-focused results, data science has allowed financial institutions to become more responsive rapidly evolving consumer needs. With the goal of understanding customers better and reducing costs while enhancing service delivery across multiple channels, this new class of analytics has quickly become a powerful driver for positive change. By shedding light on how banks can use data science to better serve their client’s interests – both now and into the future – we hope to show that sound business strategy combined with modern technology can paint a brighter financial landscape today and help make it a reality tomorrow.Â
For those looking to join this revolutionary endeavor by becoming data scientists themselves we recommend Decode Data Science With Machine Learning 1.0 by Physics Wallah. This comprehensive course offers an approachable introduction packed with expert insights into everything you need to know in order to become proficient in using data science for banks.
Data Science in Finance Overview
Data Science has emerged as a transformative force in the finance industry, revolutionizing how professionals approach data analysis, risk management, and decision-making. In finance, which deals with vast amounts of daily data, data science offers powerful tools and methodologies to extract valuable insights and drive informed strategies.
Risk management is one of the primary applications of data science in finance. Financial institutions leverage advanced analytics and machine learning algorithms to assess and mitigate risks effectively. These tools analyze historical data, market trends, and economic indicators to predict potential risks, enabling proactive decision-making and mitigation.
Data science also contributes to regulatory compliance within the finance industry. Financial institutions must adhere to stringent regulations and reporting requirements. Data science tools enable automated compliance checks, ensuring that institutions operate within the regulatory framework and avoid potential penalties.
What is a Data Scientist in the Finance Industry?
Financial data scientists are seasoned professionals possessing specialized and profound domain expertise. Operating within one of the world’s most lucrative industries, the global finance sector was among the pioneers in recognizing the immense profit potential embedded in big data.
The symbiotic relationship between banking and data analytics becomes apparent when considering their shared objective of predicting market changes to optimize investments and gain a competitive edge. Financial institutions, armed with a wealth of information spanning market metrics, transaction data, and detailed customer profiles, find a seamless integration with the predictive nature of data analytics.
However, the colossal volume of big data amassed by the finance industry presents a formidable challenge. The primary dilemma revolves around effectively harnessing unstructured data—information that lacks organization and a coherent model.Â
Complicating matters further is the intrinsic complexity of the banking sector itself. Characterized by mergers, acquisitions, intricate product offerings, and evolving regulations, banking demands a level of expertise beyond the capabilities of a conventional data analyst. Guided by adept professionals, financial service providers can streamline intricate processes and dismantle the prevalent silo culture within the industry. Their role extends beyond the conventional tasks of data analysts, encompassing not only the collection, mining, and interpretation of data but also navigating the intricate landscape of a constantly evolving industry.
Also Read: How to Become a Data Scientist: 5-Step Guide
Data Scientist in Finance Roles And Responsibilities
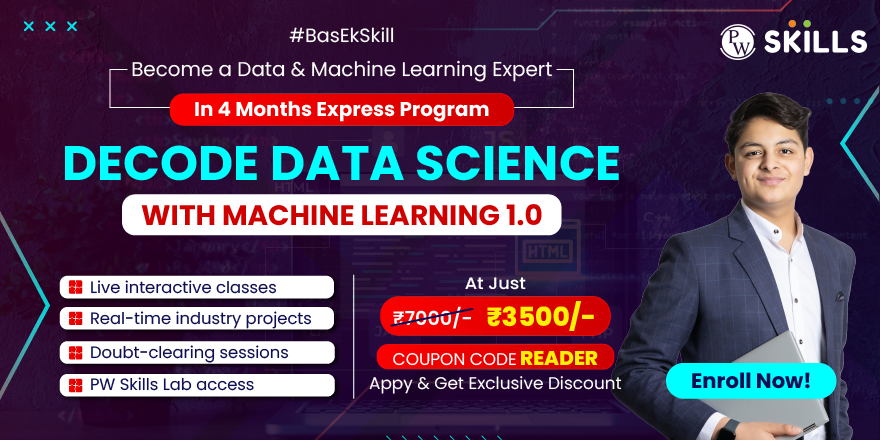
In the finance sector, the role of a Data Scientist is multifaceted, involving various responsibilities that contribute to informed decision-making and process optimization. Here are key areas of focus and responsibilities for Data Scientists in finance:
Data Analysis and Modeling:
Data Scientists leverage statistical methods and machine learning techniques to analyze extensive datasets related to financial transactions, customer behavior, market trends, and economic indicators. They develop predictive models for risk assessment, fraud detection, credit scoring, and other financial forecasting applications.
Risk Management:
Assessing and mitigating risks is a crucial responsibility. Data Scientists delve into historical and current financial data to identify patterns and trends indicating potential risks. They develop risk models to evaluate creditworthiness, assess market risks, and optimize risk-return trade-offs.
Fraud Detection:
Data Scientists design and implement algorithms and models to detect and prevent fraudulent activities in financial transactions. This involves close collaboration with cybersecurity teams to enhance the security of financial systems.
Customer Analytics:
Analyzing customer data is essential for gaining insights into behavior, preferences, and trends. Data Scientists use this information to implement personalized marketing strategies and customer segmentation and recommend products or services based on data-driven insights.
Market Research and Forecasting:
Conducting market research using financial data is a crucial responsibility. Data Scientists identify opportunities, assess market trends, and guide investment decisions. They also develop forecasting models for stock prices, interest rates, and other financial indicators.
Algorithmic Trading:
Data Scientists develop and optimize algorithmic trading strategies in this specialized area using historical market data and real-time information. This involves implementing machine learning models for automated trading and portfolio optimization.
Regulatory Compliance:
Ensuring compliance with financial regulations is critical. Data Scientists analyze data to identify potential compliance issues and implement solutions to meet regulatory requirements. Staying updated on regulatory changes and assessing their impact on financial operations is crucial.
Data Visualization and Reporting:
Data Scientists create visually compelling dashboards and reports to communicate complex financial insights to stakeholders. They collaborate closely with business teams to tailor analytical solutions to their requirements.
Cross-Functional Collaboration:
Collaboration with cross-functional teams is continuous. Data Scientists work closely with finance professionals, actuaries, IT specialists, and business analysts to integrate data science solutions into financial systems and processes.
Must Read: Everything You Need to Know About Data Science Career Path in 2023
Data Science in Finance Jobs With Salary
Here are some of the career opportunities as a data scientist in the finance industry, and we have also mentioned data science in finance salary:
Data Science in Finance Jobs With Salary | ||
Job Role | Key Roles | Salary (INR) |
Financial Analyst | Analyzing financial data, preparing reports, supporting budget processes | 6,00,000 – 12,00,000 per annum |
Big Data Analyst | Managing large datasets, extracting insights, implementing big data tech | 8,00,000 – 15,00,000 per annum |
Risk Manager | Identifying and assessing risks, developing mitigation strategies | 10,00,000 – 18,00,000 per annum |
Machine Learning Specialist | Designing and implementing ML models, collaborating with teams | 12,00,000 – 20,00,000 per annum |
Data Visualization Expert | Creating visualizations, designing dashboards, aligning with business goals | 8,00,000 – 15,00,000 per annum |
Business Intelligence Consultant | Consulting on BI strategies, designing and implementing BI solutions | 10,00,000 – 16,00,000 per annum |
Research Analyst | Conducting market research, gathering industry data, preparing reports | 6,00,000 – 12,00,000 per annum |
Natural Language Processing Specialist | Developing NLP algorithms, enhancing language understanding capabilities | 10,00,000 – 18,00,000 per annum |
To capitalize on the predictive analytics technology landscape we should all invest in mastering the fundamentals of Data Science with resources like Full Stack Data Science Pro by Physics Wallah. With this course you will equip yourself with the essential skills required to reap exceptional benefits and propel your future career success in data science and finance alike. So don’t wait any longer, join us today and unlock your potential!
How to Become A Financial Data Scientist?
Becoming a financial data scientist involves a combination of education, skill development, and practical experience. Here’s a step-by-step guide on how to embark on this career path:
Educational Background:
- Foundational Degree: Start by earning a bachelor’s degree in a relevant field such as data science, statistics, mathematics, finance, or computer science. A strong foundation in quantitative disciplines is crucial.
- Advanced Education: Pursue a master’s or Ph.D. in data science, finance, or a related field. Advanced degrees can provide in-depth knowledge and research opportunities.
Develop Core Skills:
- Analytical Skills: Gain more strength in your analytical and mathematical abilities. Working with complicated numerical data calls for financial data scientists to have a strong grasp of statistical techniques and mathematical modeling.
- Programming Languages: Become proficient in computer languages like Python and R that are frequently used in data science. These languages are necessary for machine learning, analysis, and data manipulation.
- Data Manipulation and Analysis: Master tools and libraries for data manipulation and analysis, including pandas, NumPy, and Jupyter notebooks.
- Machine Learning: Acquire knowledge of machine learning algorithms and techniques. Understand how to apply these methods to financial datasets for predictive modeling and decision-making.
Industry Knowledge:
- Finance Acumen: Develop a deep understanding of financial markets, instruments, and economic principles. This knowledge is critical for interpreting financial data and making informed decisions.
- Regulatory Environment: Stay informed about financial regulations and compliance standards. Financial data scientists often work with sensitive data and must adhere to regulatory guidelines.
Gain Practical Experience:
- Internships, Courses and Projects: Seek internships or projects that allow you to apply your skills in real-world scenarios. This practical experience enhances your resume and provides exposure to industry challenges. Enroll in data science courses like Physics Wallah courses.
- Collaborate In Competitions: Participate in data science competitions on platforms. These competitions offer a chance to solve complex problems and showcase your skills to the data science community.
Build a Strong Portfolio:
- Showcase Projects: Create a portfolio that highlights your data science projects. Include details about the problems you solved, the methodologies used, and the impact of your work.
- GitHub Repository: Use platforms like GitHub to share your code and projects. A well-maintained repository demonstrates your coding skills and project management abilities.
Networking:
- Join Data Science Communities: Connect with professionals in the data science and finance communities. Attend conferences, webinars, and meetups to expand your network.
- LinkedIn Profile: Optimize your LinkedIn profile with your educational background, skills, and projects. Engage in discussions and follow influencers in the field.
Stay Updated:
- Continuous Learning: Given the rapid evolution of technology and data science, commit to continuous learning. Stay updated on the latest tools, techniques, and industry trends.
Apply for Positions:
- Entry-Level Roles: Start applying for entry-level data science or financial analyst positions to gain initial experience.
- Specialized Roles: As you accumulate experience, target roles specifically focused on financial data science, such as quantitative analyst, risk analyst, or data scientist in financial institutions.
Decode Data Science With Machine Learning 1.0 by Physics Wallah is perhaps one of the best options available — and I heavily recommend it for anyone interested in growing their knowledge base and expertise on the subject. Why not take your first steps into this captivating field today? Who knows—maybe you’ll be the one to bring about the next big breakthrough in finance!
Skills Needed To Become A Financial Data Scientist
A typical data science role in finance often requires a Bachelor’s degree in data science, computer science, math, or statistics. Complementing academic qualifications with technical skills, such as programming languages and database architecture, through coursework or certifications enhances marketability in the competitive fintech landscape.Â
You can also download Data Science in Finance PDF to access data. Continuous learning is essential to stay abreast of advancements in the dynamic field of financial data science. Becoming a successful investment professional in data science and fintech careers necessitates combining critical skills, technical expertise, and relevant qualifications. Here’s how these skills align with the provided information:
1. Problem-Solving Mindset:
A problem-solving mindset is a crucial skill for any data science role in finance. Investment professionals need to tackle complex financial challenges using data-driven solutions.
2. Business Strategy:
Understanding business strategy is essential for aligning data analyses with broader business objectives. Investment professionals in data science should contribute to strategic decision-making through their insights.
3. Communication Skills:
Effective communication is vital in translating complex data findings into understandable insights. Investment professionals must collaborate with technical and non-technical stakeholders, making communication skills a critical asset.
4. Quantitative Analysis:
Quantitative analysis is at the core of financial data science. Investment professionals must analyze numerical data to inform investment decisions and assess market trends.
5. Python Programming:
Proficiency in Python programming is a technical skill that is highly valued in data science roles. Investment professionals use Python for data manipulation, analysis, and implementing machine learning algorithms.
6. R Programming:
R programming is another valuable language in data science. Investment professionals proficient in R can leverage its statistical capabilities for in-depth data analysis.
7. Data Visualization:
Data visualization skills are essential for conveying insights effectively. Investment professionals should be adept at using tools like Tableau or Power BI to create compelling visual representations of financial data.
8. Machine Learning Techniques:
Understanding machine learning techniques is crucial for developing predictive models, risk assessments, and other applications in finance. Investment professionals need to apply machine learning to gain insights from vast datasets.
9. Artificial Intelligence (AI):
Knowledge of AI is becoming increasingly crucial in fintech. Investment professionals may leverage AI technologies for algorithmic trading and fraud detection tasks.
10. SQL:
Proficiency in SQL is essential for managing and querying databases. Investment professionals need to extract and manipulate data efficiently for analysis.
11. Database Architecture:
Understanding database architecture is crucial for handling large volumes of financial data. Investment professionals must design and manage databases to ensure data accessibility and integrity.
Also check: 10 Domains For People Starting A Career In Data Science
Data Science in Finance Research Papers
Data science has emerged as a critical driver in shaping the landscape of finance research, leveraging technological trends to enhance decision-making processes. Researchers and scholars contribute to this dynamic field through various journals, data science in finance research topics and platforms, shedding light on theoretical aspects and practical applications.
Journal of Finance and Data Science (JFDS):
The Journal of Finance and Data Science serves as a vital interdisciplinary platform where detailed analyses of theoretical aspects in finance are presented . Researchers often contribute to this journal to share insights, methodologies, and findings that bridge the gap between finance and data science.
Big Data’s Significance in Financial Services:
Research in finance emphasizes the profound significance of big data in enterprise and technology architectures for financial services. This involves a two-step investigation process, delving into the transformative impact of big data on the financial sector. These studies provide valuable insights into the integration of data science practices in financial institutions.
Data Science in Finance and Economics (DSFE):
The international, open-access journal, Data Science in Finance and Economics (DSFE), stands as a beacon for high-quality, peer-reviewed research in the intersection of data science, finance, and economics. It acts as a repository for diverse perspectives and methodologies employed by researchers in these domains.
Journal of Financial Data Science:
The Journal of Financial Data Science focuses on research activities in data science, with a particular emphasis on big data analytics within the financial realm. Scholars contributing to this journal often highlight the importance of ensuring proper attribution when submitting their work to multiple publications, emphasizing the ethical considerations in data-driven finance research. These research papers collectively contribute to advancing the understanding of the intricate relationship between data science and finance. They explore theoretical frameworks, practical applications, and the ethical considerations that underpin the evolving landscape of financial research in the digital age.
Data Science in Finance ProjectsÂ
Here are some of the data science in finance projects you can try:
Data Science in Finance Projects | |
Project Title | Description |
Credit Scoring Model | Develop a machine learning model to assess the creditworthiness of individuals, improving risk management for lending institutions. |
Fraud Detection System | Implement advanced analytics to identify and prevent fraudulent activities in financial transactions, enhancing security and minimizing financial losses. |
Portfolio Optimization | Apply data science methodologies to optimize investment portfolios, ensuring a well-balanced and risk-adjusted approach aligned with financial goals. |
Customer Segmentation for Marketing | Utilize data science techniques to segment customers based on behavior and preferences, aiding in targeted marketing campaigns and optimizing product offerings. |
Stock Price Prediction | Develop a machine learning model to predict stock prices, incorporating time-series analysis and regression algorithms for informed investment decisions. |
Algorithmic Trading Strategy | Create algorithmic trading models using data science and machine learning to automate decision-making processes and capitalize on market inefficiencies. |
Sentiment Analysis in Financial News | Apply natural language processing to analyze sentiments in financial news and social media, helping professionals gauge market sentiment and anticipate market movements. |
Customer Churn Prediction | Develop a predictive model to identify customers at risk of churn, analyzing behavior and engagement patterns to implement effective retention strategies. |
Market Basket Analysis | Apply data science to conduct market basket analysis, identifying associations in customers’ product purchases to optimize cross-selling strategies and enhance revenue. |
Regulatory Compliance Analytics | Implement data science techniques to ensure regulatory compliance within the finance industry, detecting anomalies and enhancing reporting for regulatory requirements. |
FAQs
What skills are essential for a successful career in Data Science within finance?
Key skills for a data science career in finance include problem-solving, business strategy, communication, quantitative analysis, and proficiency in technical areas such as Python programming, R programming, data visualization, machine learning techniques, artificial intelligence, SQL, and database architecture.
How do Big Data Analysts contribute to the financial sector's growth?
Big Data Analysts in finance handle large and complex datasets to identify patterns, trends, and actionable insights. Their role is crucial in extracting meaningful information from vast data, contributing to strategic decision-making and business development.
Can Data Science help in risk management within the finance industry?
Absolutely. Data Science is instrumental in risk management within finance by developing models to assess and mitigate risks, including credit and market risks. These models aid in making informed decisions safeguarding financial institutions from potential losses.
How can Machine Learning Specialists impact financial processes?
Machine Learning Specialists in finance design and implement advanced algorithms to automate tasks, predict market trends, and optimize decision-making. Their expertise is applied to various applications, including fraud detection, credit scoring, and algorithmic trading.
What role does Data Visualization play in the financial sector?
Data Visualization in finance involves creating visual representations of complex data, making it easier for stakeholders to understand and interpret information. These visualizations contribute to strategic planning, performance analysis, and effective communication of financial insights.
How does Business Intelligence contribute to decision-making in finance?
Business Intelligence in finance focuses on transforming raw data into actionable insights. BI Consultants design and implement solutions that empower financial organizations to make informed decisions, gain a competitive edge, and navigate the dynamic financial landscape.
Is a formal education required for a career in Data Science within finance?
While a typical data science role may require a Bachelor's degree in data science, computer science, math, or statistics, additional technical skills, including programming languages and database architecture, can be acquired through coursework or supplementary certifications to enhance marketability.