Analysis of Survey Data transforms raw data into meaningful insights. By adhering to best practices, you can leverage survey findings to enhance business strategies or inform research outcomes.
Analysis of Survey Data: As a researcher, marketer, or student, have you ever struggled to make sense of all the responses from a survey you administered? You’re not alone – understanding large amounts of survey data can be an overwhelming task. Data, data everywhere – but are you making sense of it all? However, raw survey results don’t always tell the full story – real understanding comes from carefully analyzing your data.
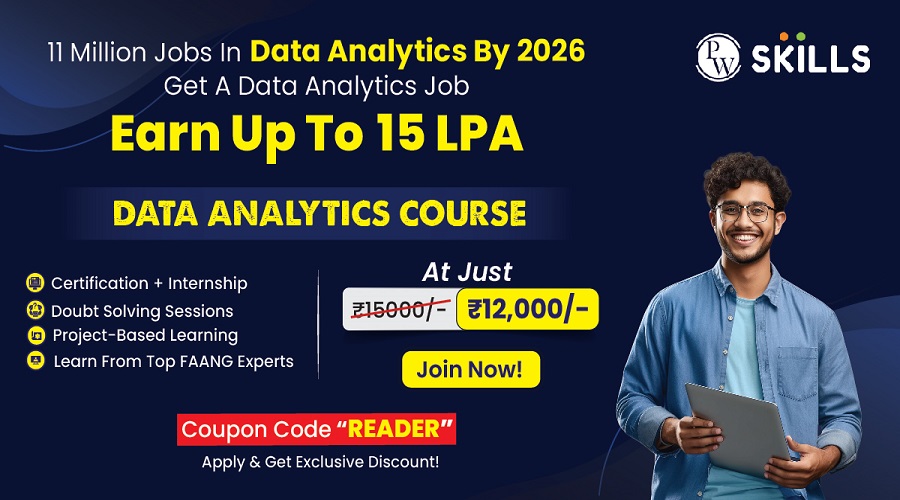
Remember to break down your data, use visual aids, and look for patterns in the responses. These strategies will help you make informed decisions and guide your next steps. But don’t stop here! Keep learning and honing your skills by enrolling in a data analytics course like the one offered by Physics Wallah.
With our experienced instructors and practical approach, you’ll be equipped with the tools and techniques needed to master survey analysis. And as a token of appreciation for being a dedicated reader, use “READER” as a coupon code to receive a discounted price for the course.
Survey Data Analysis Examples
Let’s consider a hypothetical survey about customer satisfaction with a new mobile application. The survey was distributed to 500 users, and we collected both quantitative and qualitative data. Here’s a simplified example of how you might analyze the survey data:
Quantitative Data Analysis:
- Descriptive Statistics: Begin by calculating basic statistics like mean, median, mode, and standard deviation for questions that had numerical responses, such as “On a scale of 1-10, how satisfied are you with the app?”
- Cross-Tabulation: Create cross-tabulation tables to analyze relationships between different variables. For instance, you could cross-tabulate satisfaction levels with the frequency of app usage.
- Regression Analysis: Determine if there’s a correlation between user demographics (like age, location, or occupation) and satisfaction levels. A regression model might help predict satisfaction based on these variables.
Qualitative Data Analysis:
- Thematic Analysis: Manually review open-ended responses to identify recurring themes or sentiments. For instance, common themes might include “ease of use,” “features lacking,” or “customer support.”
- Sentiment Analysis: Use text analytics tools or software to perform sentiment analysis on qualitative responses. This will help categorize feedback as positive, negative, or neutral, providing an overall sentiment score.
- Word Clouds: Generate word clouds to visualize frequently mentioned words or phrases in the qualitative feedback. This gives a quick snapshot of what users are talking about most frequently.
Also Read: Quantitative Data Analysis: Types, Analysis & Examples
How do you Analyze Survey Data? (Effective Ways)
Analyzing survey data is a crucial step in extracting meaningful insights that can drive informed decision-making and strategic planning. While gathering data is essential, interpreting it correctly is equally vital. Here’s a comprehensive guide on how to analyze survey data effectively, utilizing various techniques and best practices.
1) Comprehend the Measurement Scales
Understanding the types of measurement scales—nominal, ordinal, interval, and ratio—is foundational. Each scale serves a unique purpose and requires distinct analytical approaches:
- Nominal Scales: Utilized for qualitative data, nominal scales categorize responses without imposing any order.
- Ordinal Scales: These scales rank responses based on preferences or orders, allowing for comparative analysis.
- Interval Scales: Ideal for capturing responses within a predefined range, facilitating more nuanced analysis.
- Ratio Scales: Similar to interval scales but starting at zero, these scales provide a comprehensive quantitative assessment.
2) Prioritize Quantitative Insights
Initiate your analysis by focusing on quantitative questions that yield numerical data. Metrics like the Net Promoter Score (NPS) can offer insights into customer sentiments and brand loyalty, enabling you to identify brand advocates and areas for improvement.
3) Harness Qualitative Feedback
While quantitative data provides numerical insights, qualitative feedback offers depth and context. Analyze open-ended responses by:
- Creating visual representations to identify common themes or keywords.
- Individualizing responses to understand unique customer perspectives and expectations.
4) Implement Cross-Tabulation Analysis
Cross-tabulation facilitates a deeper understanding of the relationship between variables, especially when targeting specific demographics or segments. By segmenting data based on relevant criteria, such as age or location, you can derive more targeted insights relevant to your objectives.
5) Distinguish Between Correlation and Causation
Avoid conflating correlation with causation, as it can lead to misleading interpretations. Scrutinize data meticulously, considering external factors and variables, to draw accurate conclusions and avoid erroneous assumptions.
6) Benchmark Against Historical Data
Comparing current survey results with past data sets enables you to assess progress, identify trends, and evaluate the effectiveness of implemented strategies. By tracking key metrics over time, you can measure improvements and refine your approach continually.
7) Utilize Industry Benchmarks
Benchmarking against industry standards provides context and perspective, allowing you to gauge your performance relative to competitors and market leaders. Aligning your survey results with industry benchmarks ensures realistic goals and actionable insights.
8) Mitigate Inaccurate or Incomplete Responses
Addressing incomplete or inaccurate survey responses is crucial for maintaining data integrity. Identify and categorize inattentive respondents, such as speeders, straight-liners, slackers, and imposters, to filter out unreliable data and enhance the validity of your analysis.
Analyzing survey data is a multifaceted process that necessitates a structured approach, incorporating both quantitative and qualitative methods. By understanding measurement scales, prioritizing actionable insights, leveraging analytical techniques like cross-tabulation, and benchmarking against relevant benchmarks, organizations can derive meaningful insights to inform decision-making, optimize strategies, and drive continuous improvement.
Statistical Analysis of Survey Data
Statistical analysis of survey data involves employing various statistical techniques to analyze and interpret the collected survey responses. This analytical process aims to uncover patterns, trends, relationships, and insights from the data, enabling organizations to make informed decisions, optimize strategies, and address specific research objectives. Here’s an overview of the statistical analysis techniques commonly used in survey data analysis:
1) Descriptive Statistics:
Descriptive statistics provide a summary of the main aspects of the survey data, including measures of central tendency (mean, median, mode), variability (standard deviation, variance, range), and distribution (skewness, kurtosis). These statistics offer an initial understanding of the data’s characteristics, such as the average response, variability among responses, and distribution patterns.
2) Inferential Statistics:
Inferential statistics enable researchers to generalize findings from a sample to a larger population, assess relationships between variables, and test hypotheses. Common inferential statistical tests include:
- T-tests: Used to compare the means of two groups or assess differences between two sets of data.
- ANOVA (Analysis of Variance): Employed to compare means across multiple groups simultaneously.
- Chi-Square Test: Applied to examine the association between categorical variables and determine if observed frequencies differ significantly from expected frequencies.
- Regression Analysis: Used to identify and quantify relationships between a dependent variable and one or more independent variables, predicting the outcome based on predictor variables.
3) Correlation Analysis:
Correlation analysis assesses the strength and direction of the relationship between two continuous variables. The Pearson correlation coefficient measures the linear relationship between variables, ranging from -1 (negative correlation) to 1 (positive correlation), with 0 indicating no correlation.
4) Factor Analysis:
Factor analysis is a multivariate statistical technique used to identify underlying relationships between observed variables, uncover latent variables or factors, and reduce data dimensionality. By grouping related variables into distinct factors, researchers can simplify complex data structures and identify underlying patterns or constructs.
5) Cluster Analysis:
Cluster analysis categorizes survey respondents or variables into distinct groups or clusters based on similarities within groups and differences between groups. This technique helps segment the target population, identify distinct respondent profiles, or group similar survey items, facilitating more targeted and personalized strategies.
6) Regression Modeling:
Regression modeling encompasses various regression techniques, including linear regression, logistic regression, and multiple regression, to predict or explain the relationship between dependent and independent variables. By evaluating the impact of predictor variables on the outcome variable, organizations can identify key drivers, assess relationships, and develop predictive models.
Also Read: Analysis vs. Analytics: How Are They Different?
Analysis of Survey Data in Research
Analysis of survey data in research is a critical component that involves examining, interpreting, and making sense of the collected survey responses. It enables researchers to derive meaningful insights, identify patterns, trends, and relationships, and draw valid conclusions to address research objectives or hypotheses effectively. Here’s a comprehensive overview of the analysis of survey data in research:
1) Data Preparation:
Before conducting any analysis, researchers must prepare the survey data by cleaning, organizing, and coding the responses. This involves:
- Data Cleaning: Identifying and addressing missing, incomplete, or erroneous responses to ensure data accuracy and reliability.
- Data Transformation: Converting raw survey data into a format suitable for analysis, such as numerical coding, categorization, or scaling.
- Variable Identification: Defining variables, distinguishing between independent and dependent variables, and categorizing variables based on their type (e.g., nominal, ordinal, interval, ratio).
2) Descriptive Analysis:
Descriptive analysis involves summarizing and describing the main features of the survey data using:
- Measures of Central Tendency: Calculating mean, median, and mode to determine the average or typical response.
- Measures of Dispersion: Assessing variability using standard deviation, variance, and range to understand the spread or dispersion of responses.
- Frequency Distributions: Creating frequency tables, histograms, or bar charts to display the distribution of categorical or continuous variables.
3) Inferential Analysis:
Inferential analysis focuses on making predictions, generalizing findings, or testing hypotheses based on the survey sample data. Common inferential techniques include:
- Hypothesis Testing: Using statistical tests such as t-tests, ANOVA, chi-square tests, or regression analysis to test research hypotheses, assess differences between groups, or determine associations between variables.
- Confidence Intervals: Estimating the range within which population parameters (e.g., means, proportions) are likely to fall based on sample data.
4) Correlation and Regression Analysis:
Correlation and regression analysis help researchers understand relationships between variables, predict outcomes, and identify key predictors:
- Correlation Analysis: Using correlation coefficients to assess the strength and direction of relationships between two or more continuous variables.
- Regression Analysis: Developing predictive models to explain the relationship between dependent and independent variables, identify significant predictors, and predict outcomes based on predictor variables.
5) Factor and Cluster Analysis:
Factor and cluster analysis are advanced techniques used to identify underlying patterns, group variables or respondents, and reduce data complexity:
- Factor Analysis: Identifying latent variables or underlying constructs, reducing data dimensionality, and uncovering patterns or relationships between observed variables.
- Cluster Analysis: Segmenting respondents or variables into distinct groups based on similarities, facilitating targeted analysis, and understanding respondent segments or patterns.
Survey Data Analysis Methods
Survey data analysis serves as a critical step in understanding the collected information, drawing meaningful insights, and making informed decisions. By employing specific methods tailored to the type and structure of the survey data, researchers and analysts can effectively interpret and leverage the information gathered. Here’s a detailed exploration of various survey data analysis methods:
1) Statistical Analysis:
Statistical analysis stands as a cornerstone in survey data analysis, offering rigorous methods to examine relationships, differences, and patterns within the data. Key statistical techniques include:
- Regression Analysis: Assessing the relationship between dependent and independent variables to predict outcomes or understand associations.
- T-Test: Comparing means between two groups to determine if there are significant differences.
- Analysis of Variance (ANOVA): Evaluating differences in means across multiple groups or categories.
- Cluster Analysis: Identifying distinct groups or clusters within the data based on similarities.
- Factor Analysis: Uncovering underlying relationships between observed variables by identifying latent factors or constructs.
- Conjoint Analysis: Analyzing respondent preferences and trade-offs among different attributes or features.
2) Measurement Scales Understanding:
Recognizing the measurement scales of survey questions forms a foundational aspect of data analysis. Different scales, including nominal, ordinal, interval, and ratio scales, dictate the type of statistical tests and analyses appropriate for the data, ensuring accurate and meaningful interpretation.
3) Quantitative Questions Analysis:
Initiating the analysis with quantitative questions facilitates establishing numerical trends, patterns, and relationships within the data. By prioritizing quantitative analysis, researchers can quantify responses, calculate descriptive statistics, and derive statistical inferences to address research objectives effectively.
4) Visualization Tools:
Visual representation of survey data plays a pivotal role in conveying insights, identifying trends, and communicating findings to stakeholders. Utilizing visualization tools such as pie charts, Venn diagrams, line graphs, scatter plots, histograms, and pictograms enhances data interpretation, fosters comprehension, and facilitates decision-making processes.
5) Popular Methods Utilization:
Embracing popular methods specific to survey data analysis ensures comprehensive insights extraction. By leveraging the nine most recognized methods for survey data analysis, researchers can navigate the complexities of data interpretation, uncover hidden patterns, validate research hypotheses, and inform strategic decisions effectively.
Also Read: Learning Path to Become a Data Analyst in 2024
How to Present Survey Data
Presenting survey data in a coherent, compelling, and easily digestible manner is crucial for conveying insights, fostering understanding, and driving informed decision-making among stakeholders. By employing various methods tailored to the nature and complexity of the survey data, you can effectively communicate findings and facilitate meaningful discussions. Here’s an in-depth exploration of how to present survey data:
1) Graphical Representation:
Graphs stand as a cornerstone in presenting survey data due to their ability to simplify complex information and facilitate visual interpretation. Depending on the nature of your data, consider utilizing the following graphical representations:
- Pie Charts: Ideal for illustrating proportions and percentages, pie charts offer a clear visualization of categorical data distribution.
- Venn Diagrams: Useful for showcasing overlaps or intersections between different data sets or categories.
- Scatter Plots: Effective for displaying relationships and correlations between two variables, facilitating trend identification.
- Histograms: Perfect for representing frequency distributions and identifying data distribution patterns.
- Pictograms: Employ visuals or icons to represent data quantities, making data more relatable and engaging.
Ensure selecting the most appropriate graph type that aligns with your data characteristics and resonates with your target audience’s preferences and comprehension levels.
2) Data Tables:
Data tables serve as a structured and systematic approach to presenting numerical survey data. By leveraging tools like Excel, you can organize, categorize, and display quantitative data in a tabular format, enhancing clarity, and facilitating comparative analysis. Ensure incorporating relevant headers, footnotes, and annotations to provide context and facilitate interpretation.
3) Interactive Presentations:
Crafting interactive presentations enables you to amalgamate textual and graphical data, fostering engagement and facilitating comprehensive understanding. Begin by outlining the research objectives, methodology, and hypothesis, followed by systematically presenting survey findings, insights, and implications. Utilize visuals, animations, and infographics to enhance engagement, convey key messages, and facilitate interactive discussions.
4) Infographics:
Infographics emerge as a potent tool for presenting survey data in a visually appealing, concise, and easily consumable format. By transforming survey results into compelling visuals, statistics, and narratives, infographics enhance information retention, facilitate comprehension, and augment the aesthetic appeal of your presentations. Consider incorporating color coding, icons, and concise text to convey key findings, trends, and insights succinctly.
5) Comprehensive Reports:
For investor meetings, shareholder discussions, or detailed presentations, comprehensive reports serve as an invaluable tool for presenting survey data. While incorporating graphs, tables, and infographics, reports provide an in-depth analysis, interpretation, and contextualization of survey findings.
Ensure structuring your report systematically, including an executive summary, methodology, findings, discussions, conclusions, and recommendations. Facilitate accessibility by incorporating a table of contents, appendices, and references, ensuring stakeholders can delve deeper into specific sections or data points as required.
Common Mistakes in Analysis of Survey Data and How to Avoid Them
Analyzing survey data is a pivotal step in extracting valuable insights that can drive informed decisions, shape strategies, and inform future research endeavors. However, several common pitfalls can compromise the accuracy, reliability, and validity of your findings. Recognizing these challenges and implementing strategies to mitigate them is crucial for ensuring robust and actionable survey data analysis. Here’s a comprehensive exploration of these common mistakes and how to navigate them effectively:
1) Premature Interpretation of Results:
Common Mistake: Succumbing to confirmation bias by hastily interpreting survey results that align with preconceived notions or expectations without ensuring statistical significance.
Mitigation Strategy: Prioritize a rigorous statistical analysis approach to ascertain the validity, reliability, and significance of your findings. Emphasize the importance of a sufficiently large sample size to minimize the likelihood of skewed or coincidental results. Adopt a systematic and unbiased approach to data interpretation, emphasizing objectivity, and evidence-based conclusions.
2) Misinterpreting Correlation as Causation:
Common Mistake: Conflating correlation with causation, attributing causative relationships between variables solely based on observed correlations without considering potential confounding variables or underlying mechanisms.
Mitigation Strategy: Exercise caution and critical thinking when interpreting relationships between variables. Emphasize the importance of exploring underlying factors, mechanisms, and variables that may influence observed correlations. Encourage a comprehensive and nuanced analysis that considers potential confounders, alternative explanations, and causal pathways, ensuring accurate and informed interpretations.
3) Overlooking Nuances in Qualitative Natural Language Data:
Common Mistake: Oversimplifying the analysis of qualitative survey data, such as speech or text responses, by relying solely on superficial categorizations or failing to capture the richness, context, and intricacies of human language.
Mitigation Strategy: Leverage advanced AI solutions and machine learning algorithms capable of sophisticated sentiment analysis, contextual understanding, and nuanced interpretation of qualitative data. Prioritize tools that emulate human-like comprehension, considering context, emotion, intent, and conversational dynamics. Foster a multidimensional approach to qualitative data analysis, emphasizing depth, richness, and comprehensive understanding to extract meaningful insights effectively.
Also Read: AI and Predictive Analytics: Examples, Tools, Uses, Ai Vs Predictive Analytics
Tools for Analysis of Survey Data
Analyzing survey data requires specialized tools that can efficiently process, visualize, and interpret the collected information. Here are some commonly used tools for the analysis of survey data:
Tools for Analysis of Survey Data | |
Tool Name | Primary Features & Uses |
SPSS (Statistical Package for the Social Sciences) | – Comprehensive statistical tests
– Data management capabilities – Data visualization options |
Qualtrics | – Real-time reporting
– Cross-tabulation – Advanced statistical analysis |
SurveyMonkey | – Survey creation
– Basic analytics features – Chart generation |
Microsoft Excel | – Pivot tables
– Data visualization with charts – Basic statistical functions |
NVivo | – Qualitative data analysis
– Thematic and content analysis – Textual and multimedia data processing |
Tableau | – Interactive dashboard creation
– Data visualization – Presentation of survey findings |
R (with ggplot2) | – Statistical analysis
– Advanced visualization capabilities – Custom analyses and modeling |
Python (with Matplotlib or Seaborn) | – Data manipulation and analysis
– Visualization – Custom scripting for specific survey tasks |
If you still feel overwhelmed or want to enhance your skills further, we highly recommend enrolling in the Data Analytics course offered by Physics Wallah. Their comprehensive syllabus covers all aspects of survey data analysis and is taught by experienced professionals who are passionate about imparting their knowledge. And as a token of appreciation for being a reader of this blog post, use the “READER” coupon code to avail yourself of a special discount on the course fee.
For Latest Tech Related Information, Join Our Official Free Telegram Group : PW Skills Telegram Group
Analysis of Survey Data FAQs
What is the survey method of data analysis?
The survey method of data analysis involves collecting structured information from respondents through questionnaires or interviews. Once gathered, this data undergoes systematic examination to extract insights, trends, or patterns that can inform decision-making or research objectives.
What is the best tool to analyze survey data?
Several tools can effectively analyze survey data based on specific needs, such as SPSS, Qualtrics, SurveyMonkey, and Microsoft Excel. The "best" tool often depends on the complexity of the survey, required analytical techniques, user expertise, and desired output formats.
What is the purpose of survey analysis?
The purpose of survey analysis is to interpret collected data to understand respondent opinions, behaviors, preferences, or attitudes. By analyzing survey results, organizations or researchers can derive insights, make informed decisions, assess trends, identify patterns, and address research objectives or business challenges effectively.
What is the primary objective of analyzing survey data?
The primary objective of analyzing survey data is to extract valuable insights, patterns, and trends from the collected responses. This analysis aids in understanding respondent behaviors, preferences, opinions, and perceptions, enabling organizations to make informed decisions, shape strategies, and inform future initiatives effectively.
What are the key steps involved in analyzing survey data?
The key steps involved in analyzing survey data encompass data cleaning and preparation, defining objectives and research questions, selecting appropriate analytical techniques, conducting statistical analyses (e.g., regression analysis, t-tests, ANOVA), interpreting findings, and communicating results effectively.