Predictive analysis is the future of data analysis as it helps companies predict future trends and customer behaviour. So, learning predictive analysis is important for aspiring data analysts.
Predictive Analysis: Predictive analysis is the process through which you can predict the future events. It is mainly used by businesses that want to predict the evolving market trends and customer preferences. Through this article, you will study the intricacies involved in predictive analytics. You will also understand why learning it is important for your future.
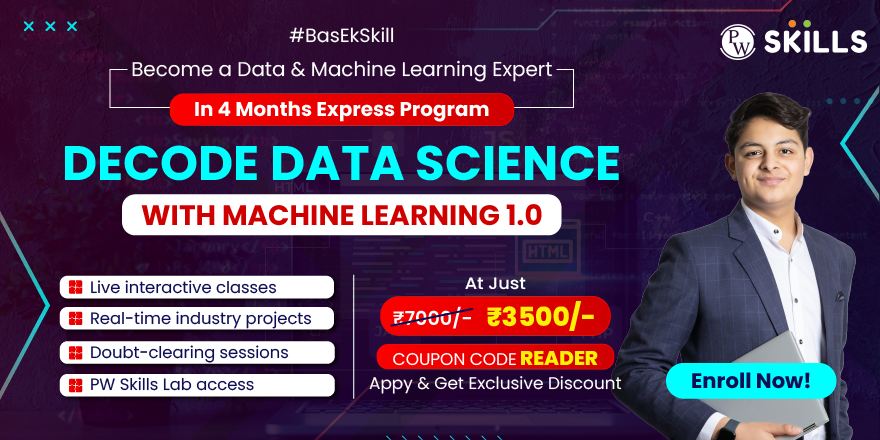
What is Predictive Analysis?
Predictive analysis is analysing historical data and trends and using it to predict future outcomes. Machine learning, artificial intelligence, data analysis, and statistical models are used to forecast the future trends. Companies that forecast events and trends accurately have a better hold over customers and business outcomes.
Also read: 6 Most-In-Demand Predictive Data Science Models in 2023
How Does Predictive Analysis Work?
Collected data is organized and pre-processed to develop predictive models. These models are trained with data to generate accurate predictions of the future. The entire process is explained through the below steps:
Step 1: Defining the problem
First, you will have to identify the problem statement i.e. reason for which you want to develop a predictive analysis model. Companies can use it to determine whether they have enough inventory for a holiday sale or not.
They can also use it to find the appropriate time to launch a new product or service. Defining the problem will enable you to select the appropriate predictive analytics model.
Step 2: Collect and Organize Data
After defining the problem statement, you will have to identify trustworthy data sources. You can obtain data from legacy systems, social media, and other reliable sources. Once you obtain the data, you can organize it using Google Big Query or other data repositories.
Step 3: Pre-processing the data
To feed quality data to the predictive model, you must pre-process it. It includes cleaning data, completing the missing points, and identification of irrelevant data. After removing the anomalies, you can expect the predictive models to generate accurate future trends.
Step 4: Development of predictive model
Finally, you can develop a predictive model using different data analytical software and tools. Regression models, decision trees, and machine learning are some of the common predictive techniques used in several industries.
Step 5: Experiment
After developing a predictive model, you must feed it with different types of data. Once the model starts generating accurate results, you can create reports and share with your stakeholders.
Recommended Technical Course
- Full Stack Web Development Course
- Generative AI Course
- DSA C++ Course
- Java+DSA 1.0 Course
- Data Analytics Course
- Data Science with ML 1.0 Course
Popular Predictive Analysis Techniques
Predictive analytics models are basically of two types viz. classification model and regression models. Classification models tend to categorize outcomes or customers into two specific categories. For example, you can use it to identify the customers that do not mind receiving marketing materials through email.
Regression models try to predict the future data which is of continuous nature. For instance, you can predict the CLTV (Customer Lifetime Value) i.e. the lifetime value generated by a customer during their relationship with your brand. Now, let’s dive deeper into the predictive analysis techniques:
-
Regression Analysis
With regression analysis, you can establish correlation between different variables in data. It is mainly used to recognize patterns in continuous data with a known distribution. The effect of one or multiple variables on each other can be studied using regression analysis. For example, how increasing or decreasing price will affect sales of a product can be studied using a regression analytics model.
Also read: Types Of Regression Analysis In Machine Learning
-
Decision Trees
Decision trees are classification techniques used to determine multiple results based on different variables. This predictive analysis model looks like a tree as each branch represents a possible choice or outcome. Decision trees can also be used to analyse datasets that have missing variables.
-
Neural Networks
Neural networks are ML (Machine Learning) models that prove to be useful in analysing complex relationships between variables. They are mostly used to establish non-linear relationships in huge datasets. You can also use them to test the outcomes of regression models and decision trees.
Why is Learning Predictive Analysis Important?
Every industry is getting competitive day-by-day. To survive the extreme competition, businesses try to understand evolving customer behaviour and trends.
Maintaining a lean inventory, making right product decisions, etc. is possible when you know how the market will behave in the distant future. Predictive analysis provides crucial marketing insights by predicting the future events. So, forecasting is a critical aspect of data analysis for most companies these days.
If you learn how to handle and use data for predictive models, you can easily get the position of a data analyst or business analyst in top companies. It will validate your potential of using raw data to predict business outcomes.
In many ways, predictive analytics is the future of data analytics. As per a study conducted by Allied Market Research, the global predictive analytics market will reach $35.45 billion USD by 2027. A growth rate (CAGR) of 21.9% make it one of the fastest growing verticals in the field of data analytics. So, ignoring this area will only affect your reputation as an expert data analyst.
Predictive analytics will be used in HR to determine common employee issues. It will help the HR teams to reduce employee churn by upskilling or providing fair compensation. Predictive analysis is already playing a key role in healthcare, retail, marketing, and other industries. So, it will be only fair to say that the demand for predictive analysts will grow in the future.
Considering the above points, you must gather more knowledge and skills related to predictive analysis. You can enroll in online courses that provide in-depth knowledge on data analysis and related concepts.
Want to be a certified data analyst? At PW Skills, we offer a wide range of data analytics courses. You can develop data analysis skills from scratch by participating in a mastering data analytics course. Apart from a highly informative and comprehensive syllabus, we provide recorded videos, practice questions, and learning resources. With our courses, you can learn popular technologies like Python, AI, Java Spring, and more. Explore our online courses now by clicking here!
Also read: Time Series Analysis in Data Science: A Step-by-Step Guide
PW Skills Provide Various Platform
FAQs
Why should I learn predictive analysis?
By learning predictive analysis, you can get more job opportunities in organizations that are keen on optimizing their campaigns and managing resources. Predictive analytics can be applied to business management, data analysis, and many other fields. So, you can shape your career by acquiring in-depth knowledge and skills related to predictive analysis.
Which skills are to be required to perform predictive analysis?
You must learn machine learning techniques, data mining, AI (Artificial Intelligence), and other emerging data science technologies. Apart from that, you must have in-depth knowledge of statistical modelling and concepts like regression analysis and decision trees.
What business problems does predictive analysis solve?
The future is uncertain, and entrepreneurs are perplexed due to this uncertainty. Predictive analysis eliminates the uncertainty hovering around market trends, customer behaviour, pricing strategies, and more. It provides a clearer picture of the future and helps businesses to take well-informed business decisions.
What is the future of predictive analytics?
In the future, technologies like AI and ML will be increasingly used in developing predictive analytics models. These technologies will continue to evolve and help companies in designing accurate predictive models.
Which industries need predictive analytics?
Almost all the industries like banking, healthcare, retail, manufacturing, and supply-chain units can benefit from predictive analysis.
How to start learning predictive analytics?
Start by defining the business objectives. Now, find the datasets which are relevant to your business and industry. Finally, choose the right software and tools to process the data and to retrieve meaningful insights.
Is it difficult to study predictive analysis?
Learning predictive analysis can be difficult, especially for the students who have little or no knowledge about data science, computers, mathematics, or statistics.
Which techniques are used in predictive analysis?
Regression analysis, neural networks, and decision trees are the popular techniques used in predictive analysis.
Which are the examples of predictive analysis in real life?
Ecommerce brands using predictive analysis to predict customer buying behaviour and pattern is a prime example of predictive analysis in real life. Companies using predictive analytics to understand customer sentiments to create better marketing strategies is also an example of predictive analytics. Netflix using predictive analytics to suggest movies and web series to the customers is also a popular example.
Why do companies use predictive analysis?
Companies use predictive analytics to reduce employee churn, improve marketing strategies, understand customer sentiments, and make accurate sales forecasts.