Data Science in Healthcare: In an era dominated by technological advancements, data science has emerged as a transformative force, particularly in the realm of healthcare. The convergence of data analytics, artificial intelligence, and machine learning has given rise to a new era of precision medicine, operational efficiency, and improved patient outcomes.
In this blog, we’ll talk about the role of Data Science in healthcare, benefits of data science in healthcare, scenario in India, and much more!
If you want to become a successful and in-demand data scientist, then a Full-Stack Data Science Course can really help you!
Why Do We Use Data Science in Healthcare?
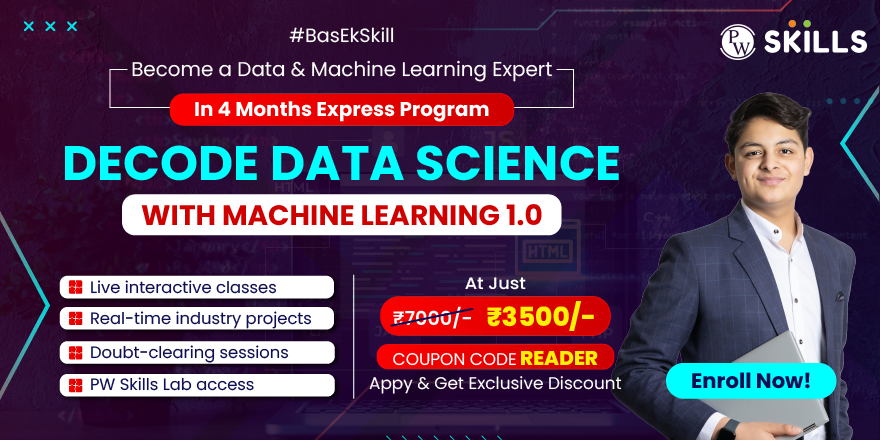
Healthcare, once reliant on traditional methods, is increasingly adopting data-driven approaches to tackle complex challenges. Healthcare data, spanning electronic health records to genomic details, requires advanced analytics for meaningful insights. Data science empowers healthcare professionals with tools for informed decisions, enhancing patient care.
Predictive analytics is a cornerstone of data science in healthcare, allowing practitioners to foresee potential health issues and intervene proactively. By analysing historical patient data, predictive models can identify patterns and trends that may indicate the likelihood of specific medical conditions. This enables healthcare providers to tailor interventions, ultimately improving patient outcomes and reducing the burden on healthcare systems.
Application and Use Cases of Data Science in Healthcare
In healthcare, data science applies broadly, impacting patient care, disease prevention, efficiency, and research.
Predictive Analytics: Anticipating Disease Outcomes
Predictive analytics stands at the forefront of data science applications in healthcare. By harnessing historical patient data, clinical records, and environmental factors, predictive models can anticipate disease outcomes. This is particularly valuable in chronic disease management, where identifying early warning signs allows healthcare providers to intervene proactively.
For example, in diabetes management, predictive analytics can analyse patient data to identify individuals at a higher risk of complications. This enables healthcare professionals to implement personalised interventions, such as lifestyle modifications or medication adjustments, to prevent adverse outcomes.
Read More: Predictive Analytics Examples: Real World Applications and Insights
Personalised Medicine: Tailoring Treatments to Individuals
Personalised medicine, enabled by data science, involves tailoring medical treatments to individual characteristics. This includes analysing genetic and molecular data to understand a patient’s unique response to medications and therapies. Data-driven insights guide healthcare professionals in prescribing treatments that are more likely to be effective and with minimal side effects. In the field of oncology, personalised medicine has seen remarkable advancements. Genetic profiling of tumours allows oncologists to identify specific mutations and recommend targeted therapies. This not only improves treatment efficacy but also reduces the likelihood of patients undergoing treatments that may not be beneficial for their particular cancer type.
Fraud Detection: Safeguarding Healthcare Systems
Beyond patient care, data science plays a crucial role in safeguarding healthcare systems from fraudulent activities. The complexity of billing processes and the sheer volume of transactions make healthcare systems susceptible to fraud. Data science algorithms can analyse billing patterns, identify anomalies, and flag potentially fraudulent claims. Healthcare fraud detection systems, powered by machine learning algorithms, learn from historical data to recognize patterns associated with fraudulent activities. This proactive approach helps prevent financial losses for healthcare organisations and ensures that resources are allocated to genuine patient care needs.
Operational Efficiency: Optimising Healthcare Processes
Data science contributes significantly to operational efficiency by optimising various healthcare processes. This involves optimising patient schedules, allocating resources, and managing the supply chain. Data-driven insights help:
- Identify bottlenecks
- Reduce wait times
- Improve overall service
For instance, data analytics can optimise hospital bed management by predicting patient admission rates and discharge patterns. This allows hospitals to allocate resources more effectively, reducing overcrowding and improving the overall patient experience.
Clinical Decision Support Systems
Data science plays a key role in crafting Clinical Decision Support Systems (CDSS). These systems aid healthcare experts by examining patient data, medical literature, and optimal approaches, delivering immediate suggestions for diagnosis and treatment. A notable example is the integration of CDSS in radiology. Machine learning algorithms can analyse medical images, assisting radiologists in identifying subtle abnormalities or patterns indicative of diseases. This not only enhances diagnostic accuracy but also expedites the reporting process.
Remote Patient Monitoring and Telehealth
The advent of remote patient monitoring and telehealth has been accelerated by data science. Wearable devices and sensors collect continuous streams of patient data, ranging from vital signs to activity levels. Data analytics processes this information in real-time, enabling healthcare providers to remotely monitor patients with chronic conditions. Telehealth platforms leverage data science for virtual consultations, triaging patients based on severity, and optimising appointment scheduling. Especially in times of global health crises, such as the COVID-19 pandemic, telehealth has proven to be a vital tool for delivering healthcare services while minimising the risk of infection.
Epidemiological Surveillance and Outbreak Prediction
Data science is crucial in epidemiological surveillance, helping public health agencies monitor the spread of diseases and predict outbreaks. By analysing diverse datasets, including social media, climate data, and healthcare records, data scientists can identify patterns indicative of potential outbreaks. During the early stages of an outbreak, such as a flu epidemic or a new infectious disease, data science enables rapid response strategies. Predictive modelling helps allocate resources, plan public health interventions, and minimise the impact of the outbreak on the population.
Genomic Data Analysis for Precision Medicine
Genomics underwent a transformative shift due to data science. Examining extensive genomic datasets helps pinpoint genetic variations linked to diseases and treatment responses. This data proves pivotal in propelling precision medicine, tailoring interventions to an individual’s genetic composition. Illustrating this potential, initiatives like the “100,000 Genomes Project” in the UK highlight the might of genomic data analysis. Through sequencing tens of thousands of genomes, researchers strive to unravel the genetic roots of diverse diseases, opening avenues for more precise and efficient treatments.
Role of a Data Scientist in Healthcare
The Skill Set and Qualifications Required
In healthcare, a data scientist’s role is diverse, demanding a distinct skill set merging technical prowess with domain expertise. Proficiency in statistics, machine learning, and programming languages like Python or R is crucial. Additionally, healthcare data scientists must possess a deep understanding of medical concepts, terminology, and regulatory considerations.
Collaborative Role in Interdisciplinary Healthcare Teams
Data scientists in healthcare rarely work in isolation. Collaborating with clinicians, researchers, and IT professionals, they bridge the gap between data analysis and practical healthcare applications. Effective communication and the ability to translate complex technical findings into actionable insights are critical in this collaborative environment.
Due to the sensitive nature of healthcare data, these professionals must be highly mindful of ethical and privacy considerations. Strict adherence to regulatory frameworks like HIPAA is indispensable. Data anonymization, encryption, and secure storage practices are integral to maintaining patient confidentiality and trust.
Benefits Of Data Science in Healthcare
In the dynamic landscape of healthcare, data science emerges as a potent catalyst for positive change. The integration of advanced analytics, artificial intelligence, and machine learning into healthcare systems brings forth a myriad of benefits that extend far beyond traditional practices.
- Improved Patient Outcomes and Care Quality:
-
-
- Precision Medicine: Data science facilitates crafting personalised treatment plans based on an individual’s genetic, lifestyle, and environmental factors. This ensures treatments align with each patient’s unique traits, boosting effectiveness and minimising adverse effects.
- Predictive Modelling for Chronic Conditions: Data science enables healthcare providers to predict the likelihood of chronic conditions, such as diabetes or cardiovascular diseases, by scrutinising historical patient data.
-
- Cost Reduction and Resource Optimization:
-
-
- Operational Efficiency: Through the optimization of healthcare processes, data science contributes to significant cost reductions. Efficient resource allocation, streamlined workflows, and the prevention of fraudulent activities all contribute to a more cost-effective healthcare system.
- Preventive Care: Predictive analytics helps identify individuals at high risk of developing costly chronic conditions. By implementing preventive measures and lifestyle interventions, healthcare costs associated with long-term treatment and hospitalizations can be substantially reduced.
-
- Accelerated Drug Discovery and Development:
-
-
- Targeted Drug Identification: Data science expedites the drug discovery process by analysing vast datasets related to molecular structures, clinical trials, and patient responses. This accelerates the identification of potential drug candidates and increases the efficiency of bringing new therapies to market.
- Personalised Treatment Approaches: Grasping the genetic and molecular foundations of diseases allows for targeted therapies. This not only heightens treatment effectiveness but also lowers the chances of adverse reactions, elevating patient safety.
-
- Enhanced Disease Prevention and Early Detection:
-
-
- Population Health Analytics: Data science facilitates the analysis of population health data to identify trends and risk factors. This information is crucial for designing targeted interventions and preventive measures, ultimately reducing the overall burden on healthcare systems.
- Early Intervention Through Predictive Modelling: Predictive models assist healthcare professionals in identifying individuals at risk of specific diseases. Early intervention based on these predictions allows for more effective treatment and management, preventing the progression of diseases to advanced stages.
-
- Streamlined Clinical Trials:
-
-
- Patient Recruitment and Eligibility: Data science streamlines the recruitment process for clinical trials by identifying eligible participants more efficiently. This not only accelerates the trial timeline but also ensures that trials include diverse patient populations.
- Real-time Monitoring: Advanced analytics enable real-time monitoring of clinical trial data, allowing for immediate identification of adverse events or positive outcomes. This enhances the overall safety and effectiveness assessment during the trial period.
-
- Enhanced Patient Engagement and Experience:
-
-
- Telehealth and Remote Monitoring: Data science contributes to the expansion of telehealth services and remote patient monitoring. These technologies not only improve access to healthcare services but also empower patients to actively participate in their own care, leading to better health outcomes.
- Personalized Health Recommendations: Analysing patient data helps create personalised health recommendations and interventions. From dietary advice to exercise regimens, these personalised plans enhance patient engagement and contribute to healthier lifestyles.
-
- Public Health Surveillance and Response:
-
-
- Disease Surveillance: Data science plays a pivotal role in monitoring the spread of infectious diseases and identifying potential outbreaks. Timely analysis of epidemiological data enables public health agencies to implement preventive measures and respond swiftly to emerging health threats.
- Vaccination Planning and Distribution: Predictive modelling assists in planning and optimising vaccination programs. This is especially crucial during pandemics, ensuring that vaccines are distributed strategically to areas at higher risk of disease transmission.
-
- Continuous Quality Improvement:
-
- Feedback Loops for Quality Metrics: Data science enables the creation of feedback loops for continuous quality improvement. By analysing clinical outcomes, patient feedback, and operational data, healthcare providers can identify areas for improvement and implement changes to enhance overall care quality.
- Benchmarking and Comparative Effectiveness Research: Comparative effectiveness research, facilitated by data science, allows healthcare providers to benchmark their practices against industry standards. This process supports evidence-based decision-making and continuous quality enhancement.
Data Science Healthcare Projects
Numerous pioneering data science initiatives greatly influence healthcare. Consider the “IBM Watson for Oncology” project: it employs AI to aid oncologists in pinpointing tailored, evidence-based cancer treatments. These projects highlight data science’s capacity to transform patient care and treatment approaches. Beyond individual projects, the successful implementation of data science in healthcare has far-reaching effects. The adoption of electronic health records (EHRs) and interoperability standards has facilitated seamless data sharing among healthcare providers. This interconnected ecosystem improves care coordination, reduces redundancies, and ultimately enhances patient outcomes.
How to Become a Healthcare Data Scientist in 2024?
Becoming a healthcare data scientist requires a solid educational foundation and a diverse skill set. A bachelor’s or master’s degree in a relevant field such as computer science, statistics, or bioinformatics is often a starting point. Additionally, proficiency in programming languages (Python, R), statistical analysis, machine learning, and domain-specific knowledge in healthcare is essential.
Certifications and Additional Training for Healthcare-Specific Data Science
Certifications can add significant value to a healthcare data scientist’s profile. Industry-recognized certifications in data science, machine learning, and healthcare-specific domains demonstrate proficiency and commitment to professional development. Additionally, staying updated on emerging technologies and trends through continuous learning is crucial in this dynamic field.
Practical Tips for Building a Successful Career
Navigating a career in healthcare data science requires a strategic approach. Networking with professionals in the field, participating in relevant conferences and workshops, and engaging with online communities can provide valuable insights and opportunities. Building a strong portfolio showcasing real-world projects and outcomes is also instrumental in securing meaningful roles in healthcare data science.
Future of Data Science in Healthcare
Emerging Trends and Technologies Shaping the Future
The future of data science in healthcare holds exciting possibilities. Emerging technologies such as quantum computing, edge computing, and advanced data visualisation tools are poised to redefine how healthcare data is processed, analysed, and utilised. These technologies promise to unlock new dimensions of understanding and innovation in healthcare analytics.
Anticipated Advancements in Healthcare Data Analytics
As healthcare data continues to grow in complexity and volume, advancements in data analytics will play a pivotal role. Integration of artificial intelligence and machine learning algorithms into everyday clinical practice, the development of more sophisticated predictive models, and the enhancement of real-time analytics capabilities are among the anticipated advancements that will shape the future of healthcare data analytics.
Also Read: The Future Scope of Data Science
Data Science in Healthcare Examples
The real-world applications of data science in healthcare are diverse and impactful, revolutionising how medical professionals approach patient care, disease prevention, and healthcare management. The following examples highlight the tangible and transformative effects of data-driven solutions in the healthcare sector.
- IBM Watson for Oncology
One of the pioneering projects in healthcare data science is IBM Watson for Oncology. This initiative harnesses the power of artificial intelligence to assist oncologists in making informed, evidence-based decisions about cancer treatment options. By analysing vast amounts of medical literature, clinical trial data, and patient records, Watson for Oncology provides personalised treatment recommendations, taking into account the unique genetic makeup of each patient. This not only enhances the precision of cancer care but also accelerates the decision-making process, crucial in the context of time-sensitive treatments.
- Google Health: Machine Learning for Early Disease Detection
Google Health is at the forefront of using machine learning algorithms for early disease detection. Leveraging advanced data analytics, Google Health analyses large datasets of medical records to identify patterns and risk factors associated with various diseases. For instance, machine learning models can predict the likelihood of diabetic complications by analysing historical patient data. Such early detection not only improves patient outcomes but also reduces the overall burden on healthcare systems by enabling timely and targeted interventions.
- Natural Language Processing at the Mayo Clinic
The Mayo Clinic, a renowned healthcare institution, has embraced natural language processing (NLP) to extract valuable information from unstructured clinical notes. NLP algorithms analyse free-text notes written by healthcare providers, extracting relevant data such as diagnoses, treatment plans, and patient outcomes. This data, once structured, contributes to a more comprehensive understanding of patient histories and supports clinical decision-making. The Mayo Clinic’s approach illustrates how innovative data science methods can unlock insights from previously underutilised sources of information.
- Fast Healthcare Interoperability Resources (FHIR) Standard
Interoperability is a key challenge in healthcare, with data residing in disparate systems that often struggle to communicate with each other. The Fast Healthcare Interoperability Resources (FHIR) standard addresses this challenge by providing a standardised framework for exchanging healthcare information. FHIR facilitates seamless data sharing among different healthcare systems and applications, promoting interoperability and improving the accessibility of patient information. This standard plays a crucial role in enhancing care coordination, reducing redundancies, and ensuring that healthcare providers have access to comprehensive and up-to-date patient data.
- Remote Patient Monitoring with Wearable Devices
The integration of wearable devices and remote patient monitoring exemplifies how data science is extending healthcare beyond traditional clinical settings. Wearable devices equipped with sensors collect continuous streams of health-related data, such as heart rate, activity levels, and sleep patterns. Data scientists analyse this information to monitor patients remotely, detect anomalies, and provide early interventions. This approach is particularly valuable for managing chronic conditions, ensuring that healthcare professionals can proactively address changes in a patient’s health status.
- Population Health Analytics for Public Health Interventions
Data science plays a vital role in population health analytics, where large datasets are analysed to identify public health trends and inform interventions. For example, during disease outbreaks, data scientists analyse demographic data, travel patterns, and clinical information to model the spread of the disease and predict areas at higher risk. This information guides public health agencies in deploying resources, implementing targeted interventions, and minimising the impact of the outbreak.
Data Science in Healthcare in India
The adoption of data science in healthcare is not confined to developed nations; developing countries like India are also witnessing transformative changes. The integration of electronic health records, telemedicine initiatives, and data-driven public health interventions are contributing to a more comprehensive and efficient healthcare ecosystem. While the potential benefits of data science in Indian healthcare are vast, challenges such as data quality, interoperability, and resource constraints need to be addressed. Collaborative efforts between government bodies, healthcare institutions, and the private sector can unlock the full potential of data science, offering solutions tailored to the unique healthcare landscape in India.
Must Read: Finding the Best Data Science Courses: A Comprehensive Guide
Conclusion
The integration of data science in healthcare is a paradigm shift that holds immense promise for the future. From improving patient outcomes and care quality to revolutionising drug discovery and development, data science is reshaping the healthcare landscape. As we navigate the complexities of this transformative era, the role of data scientists in healthcare becomes increasingly pivotal. By embracing new technologies, staying abreast of emerging trends, and fostering interdisciplinary collaboration, we can unlock the full potential of data science to create a healthier and more connected world.
Launch your data science career with confidence. Master the art of data science with our comprehensive Full Stack Data Science Pro course. Gain hands-on experience with industry-standard tools and technologies. Enroll Now!
FAQs
How does data science contribute to mental health care?
Data science plays a pivotal role in mental health care by analysing diverse datasets, including social media interactions and wearable device data, to identify patterns indicative of mental health conditions. Predictive analytics can help in early intervention and personalised treatment plans, contributing to improved mental health outcomes.
Can data science address healthcare disparities in underserved communities?
Yes, data science can play a crucial role in addressing healthcare disparities by analysing demographic and health data to identify gaps in access and outcomes. By understanding these disparities, policymakers and healthcare providers can implement targeted interventions to improve healthcare equity in underserved communities.
How is data science shaping the future of clinical trials?
Data science is transforming the landscape of clinical trials by optimising participant recruitment, predicting patient responses to treatments, and expediting the drug development process. Advanced analytics help researchers design more efficient and patient-centric trials, accelerating the pace of bringing innovative treatments to market.
Are there ethical considerations in using data science for healthcare?
Absolutely. Ethical considerations in healthcare data science include maintaining patient privacy, ensuring informed consent for data usage, and addressing biases in algorithms. Data scientists must adhere to strict regulatory frameworks, such as HIPAA, and prioritise ethical practices to build trust and safeguard patient confidentiality.
How can data science contribute to public health emergency preparedness?
Data science plays a critical role in public health emergency preparedness by analysing data from various sources, such as social media, climate patterns, and historical health records. This enables early detection of potential outbreaks, optimization of resource allocation, and rapid response strategies to mitigate the impact of public health crises.