Real life examples of data science applications in various industries are discussed below in this article. Find all details on data science applications and examples 2024!
Real Life Examples of Data Science Applications: Data science is a rapidly expanding field with endless potential for real-life applications. From personalized recommendations to predicting weather patterns, data science is shaping the way we live, work, and interact with the world around us.
In this article, we will discuss real-life examples of data science applications. And what better way to learn data science than with Decode Data Science With Machine Learning 1.0 course by Physics Wallah – an all-in-one course and app that will help you decode this complex field in a simplified manner. Start your journey towards success with this course today! Remember, as readers, it’s not enough to just read about something – take that step forward and invest in your future by gaining knowledge through this amazing resource! Use the “READER” coupon to avail instant discounts on PW courses!
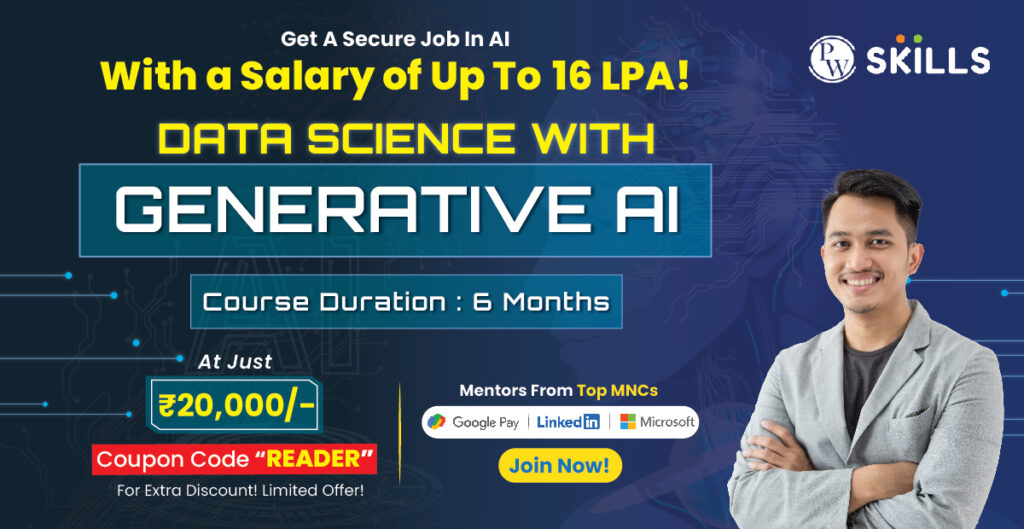
Real life Applications of Data Science Definition
Data science finds its real-life applications in various sectors, serving as a transformative force in optimizing processes and making informed decisions based on data-driven insights. A prime example can be witnessed in the manufacturing industry. Here, manufacturers leverage data science techniques to predict product demand accurately.
What is Data Science With Example?
Data science is a multidisciplinary field that combines various disciplines to extract meaningful insights from data for business purposes. Data science integrates math, statistics, specialized programming, advanced analytics, artificial intelligence (AI), and machine learning.
The primary objective is to analyze large volumes of data to derive actionable insights that can drive decision-making and strategy in businesses.
Example: In a business context, data science can be used to analyze customer purchase histories, website interactions, and feedback to identify trends in consumer behavior. By analyzing this data, businesses can make informed decisions about marketing strategies, product development, and customer engagement.
Top 10 Applications of Data Science
Data science has permeated various sectors, bringing transformative changes and insights. Here are the top 10 applications and data science applications examples:
1) Healthcare and Medicine:Â
Predictive analytics aids in forecasting patient admissions, personalized medicine, disease outbreak detection, and improving patient outcomes through data-driven decision-making.
2) E-commerce and Retail:Â
Recommendation systems, demand forecasting, customer segmentation, and price optimization are key areas where data science enhances user experience and boosts sales.
3) Banking and Finance:Â
Fraud detection, risk management, algorithmic trading, credit scoring, and customer segmentation are pivotal applications that optimize financial operations and mitigate risks.
4) Manufacturing and Supply Chain:Â
Predictive maintenance, inventory optimization, supply chain analytics, and demand forecasting are leveraged to enhance efficiency and reduce operational costs.
5) Transportation and Logistics:
Route optimization, predictive maintenance for vehicles, demand forecasting, and real-time fleet management utilize data science for efficient operations.
6) Energy Sector:Â
Predictive maintenance of equipment, energy consumption forecasting, grid management, and renewable energy optimization are some areas where data science plays a crucial role.
7) Telecommunications:Â
Customer churn prediction, network optimization, targeted marketing, and personalized service recommendations are enhanced through data-driven strategies.
8) Agriculture:Â
Precision farming, crop yield prediction, soil health monitoring, and pest prediction leverage data science to optimize agricultural practices and increase yields.
9) Entertainment and Media:Â
Content recommendation systems, audience segmentation, sentiment analysis, and personalized advertising are driven by data science to enhance user engagement and revenue.
10) Government and Public Policy:Â
Crime prediction, urban planning, public health monitoring, and policy decision-making utilize data science to address societal challenges effectively.
Data science applications span a broad spectrum of industries, offering insights, optimizing operations, and driving innovation across various sectors.
Applications and Examples of Data Science in AI
Artificial Intelligence (AI) is a subset of data science, focusing on the development of algorithms and models that can perform tasks typically requiring human intelligence. Here are some real-life examples of data science applications within the realm of AI:
1) Natural Language Processing (NLP):
- Chatbots and Virtual Assistants: Tools like Siri, Alexa, and Google Assistant use NLP to understand and respond to user queries.
- Sentiment Analysis: Analyzing text from social media, customer reviews, or feedback forms to gauge public sentiment towards products, services, or events.
2) Machine Learning Algorithms:
- Recommendation Systems: Platforms like Netflix and Amazon use algorithms to suggest movies, shows, or products based on user behavior and preferences.
- Image and Speech Recognition: Applications like facial recognition for security purposes, image tagging on social media, and voice commands in smartphones utilize advanced machine learning models.
3) Deep Learning:
- Neural Networks: Used in various applications such as image and speech recognition, autonomous vehicles, and medical diagnoses. For example, CNNs (Convolutional Neural Networks) are widely used for image classification tasks.
- Generative Adversarial Networks (GANs): Used in creating realistic images, videos, or audio. For instance, in video game design or movie production for special effects.
4) Reinforcement Learning:
- Gaming: AI agents trained using reinforcement learning can play complex games like Chess, Go, or Dota 2, surpassing human capabilities.
- Robotics: Autonomous robots in manufacturing or healthcare settings that can learn and adapt to new environments and tasks.
5) Predictive Analytics:
- Healthcare: Predicting patient outcomes, identifying potential diseases from medical images, or optimizing treatment plans using AI-driven predictive models.
- Financial Markets: Forecasting stock prices, detecting fraudulent transactions, or automating trading strategies using AI algorithms.
6) AI in Autonomous Vehicles:
- Self-driving Cars: Using sensors, cameras, and AI algorithms to navigate roads, detect obstacles, and make real-time decisions to ensure safety and efficiency.
7) Personalized Marketing:
- Customer Segmentation: Using AI-driven models to segment customers based on behavior, preferences, and purchase history to deliver personalized marketing campaigns.
8) Cybersecurity:
- Anomaly Detection: AI algorithms can detect unusual patterns or behaviors within networks, identifying potential security threats or breaches in real-time.
These are just a few real-life examples of data science applications showcasing how data science techniques and methodologies are integrated into various AI applications, driving innovation, efficiency, and value across industries. Dive deep into the transformative world of Generative AI with Physics Wallah’s specialized course. This program is meticulously crafted to provide participants with both theoretical understanding and hands-on experience in the domain of Generative AI.
Application and Example of Data Science Class 10
Data science might sound like an advanced concept, but its fundamental principles can be introduced and understood even at a Class 10 level. Here’s a simplified application and example of data science tailored for a Class 10 understanding:
Application: Analyzing School Exam Results
Example Scenario:
Imagine a Class 10 batch taking their final exams. The school wants to analyze the performance of students in different subjects to identify areas of improvement and excellence.
Steps Involved:
- Data Collection: Gather exam scores of all Class 10 students in subjects like Mathematics, Science, English, and Social Studies.
- Data Cleaning: Ensure that the collected data is accurate. Remove any inconsistencies or errors, like missing scores or incorrect entries.
- Data Analysis:
- Average Scores: Calculate the average score for each subject to determine the overall class performance.
- Subject-wise Analysis: Identify subjects where the average score is below or above a certain benchmark (e.g., average score below 60% indicates a need for improvement).
- Individual Performance: Rank students based on their scores in different subjects to recognize high achievers and those needing additional support.
- Visualization: Create simple bar graphs or pie charts to visually represent the performance of students in various subjects. This makes it easier to understand which subjects need more attention.
- Recommendations: Based on the analysis, provide recommendations such as:
- Introducing extra classes or tutoring sessions for subjects where performance is below average.
- Recognizing and rewarding students who excel in particular subjects.
- Adjusting teaching methods or resources based on areas identified for improvement.
Outcome:
By applying data science principles like data collection, cleaning, analysis, and visualization, the school can gain insights into the academic performance of Class 10 students. This information helps educators make informed decisions, tailor teaching methods, and provide necessary support to ensure students achieve their best potential.
Application of Data Science in Python
Python is one of the most popular programming languages for data science due to its simplicity, versatility, and the availability of numerous libraries and tools tailored for data analysis and visualization. Here’s how data science is applied using Python:
1) Data Collection:
- Web Scraping: Python libraries like Beautiful Soup and Scrapy allow for the extraction of data from websites.
- APIs: Python can interact with various APIs (Application Programming Interfaces) to fetch data from platforms like Twitter, Reddit, or financial markets.
2) Data Cleaning and Preprocessing:
- Pandas Library: One of the core libraries in Python for data manipulation. It’s used for tasks like handling missing data, removing duplicates, and transforming data formats.
- Data Normalization: Techniques like scaling and standardization are applied using tools like scikit-learn.
3) Data Analysis and Visualization:
- Data Analysis: Python offers libraries like NumPy for numerical computations and Pandas for data manipulation.
- Data Visualization: Libraries such as Matplotlib, Seaborn, and Plotly help in creating visual representations like histograms, scatter plots, heatmaps, and more.
4) Machine Learning:
- Scikit-learn: A popular Python library for implementing various machine learning algorithms like regression, classification, clustering, and dimensionality reduction.
- TensorFlow & PyTorch: These are frameworks primarily used for deep learning tasks, which are a subset of machine learning focused on neural networks.
5) Natural Language Processing (NLP):
- Python, with libraries like NLTK (Natural Language Toolkit) and spaCy, can process and analyze large amounts of textual data, enabling tasks like sentiment analysis, text classification, and entity recognition.
6) Big Data:
- Apache Spark with PySpark: For handling large-scale data processing tasks, Python can interface with Spark using the PySpark library, allowing distributed computing capabilities.
7) Web Development and Deployment:
- Python frameworks like Django and Flask can be used to create web applications that display data visualizations or provide machine learning predictions. Once developed, these applications can be deployed using platforms like Heroku or cloud services such as AWS or Google Cloud.
Also Read: Top 10 AI Companies in India
Applications of Data Science in Engineering
Data science has permeated various sectors, and engineering is no exception. By leveraging advanced analytical techniques, machine learning algorithms, and big data processing, engineers can derive valuable insights, optimize processes, and drive innovation across multiple domains within the engineering realm. Here are some notable applications:
1) Predictive Maintenance:
In industries like manufacturing and aerospace, data science enables predictive maintenance of machinery and equipment. By analyzing sensor data and performance metrics, engineers can anticipate equipment failures before they occur, minimizing downtime, reducing maintenance costs, and enhancing operational efficiency.
2) Design Optimization:
Data science facilitates design optimization processes by enabling engineers to simulate and analyze complex systems, structures, and components. Through iterative modeling and analysis, engineers can refine designs, improve performance metrics, and expedite the development cycle of products ranging from automobiles to consumer electronics.
3) Energy Efficiency and Sustainability:
Data science plays a pivotal role in enhancing energy efficiency and promoting sustainability within the engineering sector. By analyzing energy consumption patterns, optimizing resource utilization, and implementing renewable energy solutions, engineers can design eco-friendly infrastructures and systems that mitigate environmental impact.
4) Supply Chain Optimization:
Data science tools and techniques empower engineers to optimize supply chain operations, streamline logistics, and enhance inventory management. By analyzing demand forecasts, supplier performance, and distribution networks, engineers can design efficient supply chain systems that minimize costs, reduce lead times, and improve service levels.
5) Structural Health Monitoring:
In civil engineering and infrastructure projects, data science facilitates structural health monitoring by analyzing sensor data, monitoring vibrations, and assessing structural integrity. By implementing real-time monitoring systems and predictive analytics, engineers can detect anomalies, mitigate risks, and ensure the longevity and safety of critical infrastructure.
6) Quality Control and Process Optimization:
Data science enables engineers to implement robust quality control mechanisms and optimize manufacturing processes. By analyzing production data, identifying defects, and optimizing process parameters, engineers can enhance product quality, reduce waste, and optimize throughput in manufacturing facilities.
Data Science Applications in Sports
The realm of sports has embraced data science with open arms, leveraging advanced technologies to refine player performance, enhance strategic decision-making, and improve overall team dynamics. The integration of data science tools and techniques has not only revolutionized player training and game strategy but also elevated fan engagement and broadcasting experiences. Here are some intriguing applications shaping the sports landscape:
1) Player Recruitment and Talent Identification:
Inspired by the groundbreaking Moneyball approach, sports franchises are harnessing data analytics to identify undervalued players and optimize team compositions. By analyzing comprehensive player statistics, performance metrics, and advanced analytics, teams can unearth hidden talents and maximize budget efficiency.
2) Performance Analysis and Injury Prevention:
Wearable sensor technology, such as WHOOP, is enabling sports organizations to monitor athletes’ physical data, including resting heart rate, sleep cycles, and respiratory rates. By analyzing these vital metrics, teams can optimize training regimes, minimize injury risks, and ensure athletes perform at their peak.
3) Game Strategy and Tactical Insights:
Advanced AI systems, like Trace, are revolutionizing game analysis by providing coaches with comprehensive performance metrics and detailed game footage. By capturing crucial moments, analyzing player movements, and identifying tactical nuances, coaches can formulate effective strategies, adapt to opponent tactics, and gain a competitive advantage.
4) Fan Engagement and Personalized Experiences:
Data science is enhancing fan engagement by tailoring personalized experiences, optimizing ticket pricing strategies, and driving targeted marketing campaigns. By analyzing fan behavior, consumption patterns, and engagement metrics, sports organizations can enhance fan loyalty, maximize revenue, and create immersive viewing experiences.
5) Broadcasting and Media Optimization:
Media companies are leveraging data science to enhance sports coverage, optimize content delivery, and personalize viewer experiences. By analyzing viewer preferences, consumption patterns, and engagement metrics, broadcasters can tailor content delivery, enhance viewer engagement, and maximize advertising revenue.
Data Science Applications in Healthcare Industry
The connection of data science with healthcare has ushered in transformative changes, enhancing patient care, diagnosis accuracy, treatment personalization, and administrative efficiency. From predictive analytics to deep learning algorithms, data science tools are reshaping the medical landscape. Let’s delve into some groundbreaking applications:
1) Real-Time Disease Tracking:
A pivotal moment occurred in 2008 when Google pioneered real-time disease tracking using flu-related search data. While the initial Google Flu Trends faced challenges, it highlighted the potential of leveraging large datasets for public health insights. Today, institutions like the CDC employ advanced algorithms to monitor and predict disease outbreaks, facilitating timely interventions.
2) Cancer Detection and Diagnosis:
Tech giants like Google continue to innovate in healthcare. The LYNA tool, developed by Google, exemplifies the power of machine learning in healthcare. By identifying breast cancer tumors that metastasize to lymph nodes with remarkable accuracy, LYNA demonstrates how AI can assist clinicians in early and precise cancer detection, potentially saving lives.
3) Reproductive Health Monitoring:
Apps like Clue harness the capabilities of data science to provide women with insights into their menstrual cycles and reproductive health. By analyzing diverse metrics, including mood and physical symptoms, these platforms offer personalized predictions and alerts, empowering individuals to make informed decisions about their health.
4) Personalized Treatment Strategies:
Companies like Oncora leverage machine learning to derive insights from vast datasets, enabling healthcare providers to tailor treatment plans for cancer patients. By analyzing historical data on diagnoses, treatments, and outcomes, algorithms can recommend personalized chemotherapy and radiation regimens, optimizing therapeutic outcomes and minimizing side effects.
5) Enhancing Clinical Trials:
Veeva, a prominent player in the healthcare software landscape, utilizes data science to streamline clinical trials. Through tools like Vault EDC, the company facilitates data cleaning, ensuring accuracy and integrity in clinical trial findings. Such advancements expedite the research process, enabling faster approvals and enhanced patient safety.
Also Read: Human Intelligence vs. Artificial Intelligence: Top Differences
Data Science Examples in Business
Data science has become an indispensable tool for modern businesses, enabling organizations to extract valuable insights, optimize operations, drive innovation, and gain a competitive edge in the marketplace. Here are some compelling real-life examples of data science applications illustrating how businesses across various industries are leveraging data science:
1) Customer Segmentation and Personalization:
Retailers like Amazon and Walmart utilize data science to segment their customer base and deliver personalized shopping experiences. By analyzing customer behavior, preferences, and purchase history, businesses can tailor product recommendations, promotional offers, and marketing campaigns to individual consumer preferences, enhancing customer satisfaction and loyalty.
2) Predictive Analytics and Forecasting:
Financial institutions, including banks and insurance companies, leverage predictive analytics to assess credit risk, detect fraudulent activities, and optimize investment strategies. By analyzing historical data, market trends, and economic indicators, businesses can make informed decisions, mitigate risks, and capitalize on emerging opportunities.
3) Supply Chain Optimization:
Manufacturing and logistics companies utilize data science to optimize supply chain operations, improve inventory management, and enhance operational efficiency. By analyzing real-time data, demand forecasts, and supplier performance metrics, businesses can streamline production processes, minimize costs, and ensure timely delivery of products and services.
4) Marketing Campaign Optimization:
Digital marketers leverage data science to optimize marketing campaigns, maximize ROI, and enhance customer engagement. By analyzing customer behavior, campaign performance metrics, and market trends, businesses can identify target audiences, optimize ad placements, and allocate marketing budgets more effectively.
5) Product Development and Innovation:
Technology companies, such as Google and Apple, harness data science to drive product development, innovation, and market expansion. By analyzing user feedback, usage patterns, and competitive landscapes, businesses can identify market gaps, prioritize feature enhancements, and develop products that resonate with target audiences.
6) Human Resources and Talent Management:
HR departments utilize data science to optimize talent acquisition, retention, and development strategies. By analyzing employee performance metrics, engagement surveys, and turnover rates, businesses can identify top-performing candidates, address workforce challenges, and foster a culture of continuous learning and development.
Data Science Applications in Gaming
The gaming industry has experienced exponential growth over the years, reaching a valuation of approximately $195.65 billion in 2021 and projected to expand further by nearly 13 percent by 2030. This surge can be attributed in part to the strategic integration of data science and artificial intelligence (AI) technologies, which have revolutionized game development, user experience, and business operations. Here are some insightful examples illustrating how data science is transforming the gaming landscape:
1) Enhancing Online Gaming Experience:
Leading gaming companies like Activision Blizzard, known for iconic titles such as Call of Duty and World of Warcraft, leverage big data analytics and machine learning algorithms to enhance the online gaming experience. By analyzing player behavior and game telemetry data, these companies can detect and mitigate issues such as empowerment and power boosting, ensuring fair gameplay and enhancing user satisfaction.
2) Personalizing In-Game Recommendations:
Game studios like 2K Games utilize data science to analyze player gameplay patterns and preferences, enabling them to provide personalized recommendations and suggestions to improve the gaming experience. By extracting insights from gaming data, these companies can tailor game dynamics, challenges, and rewards to align with individual player preferences, thereby enhancing engagement and retention.
3) Monitoring and Optimizing Business Metrics:
Platforms like Unity, which empower developers to create interactive 3D content and games, leverage data science to monitor key business metrics and inform data-driven decision-making processes. By analyzing game performance, user engagement, and monetization metrics, companies can optimize product development strategies, enhance user acquisition and retention, and maximize revenue generation opportunities.
4) AI-Driven Game Design and Development:
AI technologies are increasingly being integrated into game design and development processes to create dynamic and immersive gaming experiences. From generating realistic environments and characters to designing intelligent opponents and adaptive gameplay scenarios, AI-powered algorithms enable developers to push the boundaries of creativity and innovation in the gaming industry.
Also Read: 30 Best Data Science Books to Read in 2024
How is Data Science used in day-to-day life?
Data science has become an integral part of our day-to-day lives, influencing various aspects of our routines and interactions. Here are some common ways in which data science is used in everyday life:
- Platforms such as Netflix, Spotify, and Amazon utilize data science algorithms to analyze user preferences and behavior, enabling them to provide personalized recommendations for movies, music, products, and content tailored to individual tastes and preferences.
- Data science plays a crucial role in healthcare, facilitating predictive analytics, personalized medicine, disease detection, and treatment optimization. Wearable devices and health apps analyze user data to monitor vital signs, track fitness metrics, and provide insights for maintaining a healthy lifestyle.
- Social media platforms like Facebook, Instagram, and Twitter leverage data science algorithms to analyze user interactions, preferences, and content engagement patterns. This enables them to optimize content feeds, recommend relevant content, target advertisements, and detect and mitigate harmful activities such as misinformation and spam.
- Banks, financial institutions, and fintech companies utilize data science for fraud detection, risk assessment, credit scoring, investment analysis, and personalized financial recommendations. These algorithms analyze transaction data, user behavior, market trends, and economic indicators to enhance security, streamline operations, and improve customer experiences.
- Ride-sharing platforms like Uber and Lyft utilize data science to optimize route planning, pricing strategies, driver allocation, and customer experiences. GPS and navigation systems analyze traffic patterns, user locations, and historical data to provide real-time navigation, estimate arrival times, and optimize travel routes.
- Online retailers and e-commerce platforms leverage data science for inventory management, demand forecasting, customer segmentation, personalized marketing, and product recommendations. These algorithms analyze purchase history, browsing behavior, product preferences, and market trends to optimize pricing strategies, inventory levels, and promotional campaigns.
- The entertainment industry utilizes data science for content recommendation, audience segmentation, user engagement analysis, and content optimization. Streaming platforms, gaming companies, and content creators analyze user interactions, viewing patterns, and feedback to tailor content, enhance user experiences, and optimize monetization strategies.
- Educational institutions, e-learning platforms, and edtech companies utilize data science for personalized learning, student performance analysis, adaptive learning pathways, and educational resource optimization. These algorithms analyze student data, learning preferences, performance metrics, and feedback to tailor educational experiences, identify areas for improvement, and optimize learning outcomes.
“Decode Data Science with ML 1.0 course” by PW offers a foundational exploration into the realms of Python programming and machine learning. Tailored for those enthusiastic about machine learning, this course serves as a stepping stone for individuals aiming to integrate Python’s capabilities with the transformative potential of machine learning.
Real- Life Examples of Data Science Applications - FAQs
What are real-life examples of data science applications in healthcare?
Real-life examples of data science applications in healthcare are predictive analytics, where algorithms analyze historical patient data to predict potential health risks, optimize treatment plans, and improve patient outcomes.
How is data science used in the retail industry?
In the retail industry, data science is used for various applications such as demand forecasting, customer segmentation, personalized marketing, and inventory optimization.
Can you provide an example of data science in transportation?
A notable example of data science in transportation is ride-sharing platforms like Uber and Lyft, which utilize data science algorithms to optimize route planning, pricing strategies, driver allocation, and customer experiences.
How does data science contribute to financial services?
In the financial services sector, data science plays a critical role in various applications such as fraud detection, risk assessment, credit scoring, investment analysis, and personalized financial recommendations.
What are data science 5 applications?
Data science applications include:
Predictive Analytics in Healthcare.
Demand Forecasting in Retail.
Route Optimization in Transportation.
Fraud Detection in Financial Services.
Content Recommendation in Entertainment & Gaming.